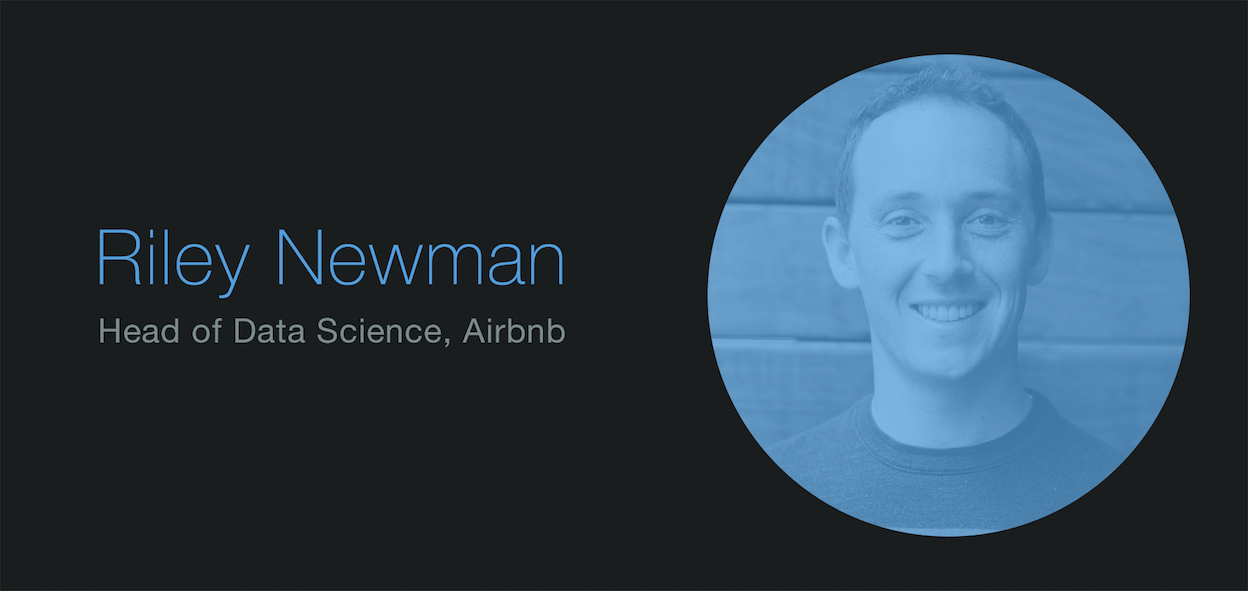
Riley Newman on data science for startups
When Riley Newman joined Airbnb nearly six years ago, he was one of 10 employees and working from the co-founders’ apartment.
Today he leads a team of 70-plus data scientists, analysts, and engineers, who are challenged with empowering more than 2,000 Airbnb employees with global insights.
I recently caught up with Riley to chat about why it’s crucial for startups to invest in data early, the difficulties of keeping data accessible at scale, how his field’s findings represent the voice of the customer, and more.
If you like what you hear, check out more episodes. You can subscribe on iTunes, Stitcher, or SoundCloud, or grab the RSS feed.
What follows is a lightly edited transcript of our conversation. Short on time? Here are six quick insights from our chat:
- It’s important that early stage startups build a strong data culture early. In many cases those insights are the difference between shutdown and success.
- Data represents a decision a customer makes. The role of a data science team is to keep the company in touch with those decisions when it’s no longer scalable to speak with every user.
- Data scientists are only as impactful as the context they have into problems they’re meant to solve. This is why Airbnb data scientists are embedded with engineers, designers, and project managers.
- As he’s scaled his own team, Riley has put as much weight on a data scientist’s ability to communicate and tell a story with their work as the technical rigor behind it.
- As a company grows, data scientists must think about how their work scales internally and make sure employees across the company have access to and understand how to use data.
- A key to data democratization: investment in infrastructure and tools surrounding data. This empowers the company and ensures the data team is able to focus on the highest impact work.
Adam Risman: Welcome to the show, Riley. For the sake of our listeners could you quickly introduce yourself and give us a quick synopsis of your career trajectory and how you got to where you are today?
Riley Newman: I run the data science team at Airbnb, and I’ve been here for about six years. I was the original analyst hired into the company, and that was before the term “data science” or crazes like big data really took off.
When I joined Airbnb it was very small. There were just a few people working out of the founders’ apartment. Obviously we’ve grown a lot over the past six years. Alongside helping to foster that growth I’ve built the data science function in the company, which is now roughly 60 data scientists and analysts, 10 data engineers, and a few others.
Prior to Airbnb I worked in a consulting firm with a group of economists. Before that I completed a Masters in Economics at Cambridge. My career arc has been relatively quantitative, but it began in the field of economics and has since moved into the application of theory within a business context.
Early Airbnb employees work from the co-founders’ apartment in San Francisco (photo: co-founder Brian Chesky).
Adam: There’s no single path into data science, but yours was particularly interesting. How does your previous experience help you look at problems differently in your day-to-day at Airbnb?
Riley: To your point, data science is a field that’s defining itself. There’s no standard path. Many of the people that we have brought into the team come from relatively quantitative backgrounds. I think my background in economics actually lends itself to a lot of the problems we’re focused on at Airbnb. This was especially true in the early days when thinking about how to get a two-sided marketplace off the ground. There’s a lot of theory that lends itself to supply and demand, thinking about guests’ and hosts’ experiences, and the match between the two.
One of the initial attractions for me coming into the company was the intellectual problem that Airbnb faced. As the company has scaled and our product has reached a greater level of sophistication, the types of people that we hire have started to skew a little bit more toward computer science and statistical backgrounds – thinking about problems like machine learning. Maybe distinct from other tech companies, we’ve also brought in a lot of people from the social sciences, and they’ve been really impactful.
The case for early data adoption
Adam: In the early days, you were one of 10 employees and working from the co-founders’ apartment. Do you think all startups should invest in data and analytics that early, or was this an exception? What were the benefits of you being there so early in the business’ life?
Riley: Nowadays it’s more conventional wisdom that you should bring a data-oriented team within the company as soon as possible.
As I mentioned the term data science and big data hadn’t really taken off yet, so it was especially anomalous that the founders brought me in that early. But it showed a lot of foresight that they were amassing data and they wanted to ensure there was a strong rudder on the ship. We weren’t just going wherever the wind blew us.
Airbnb co-founders Joe Gebbia, Nathan Blecharczyk, and Brian Chesky made data investment a priority at an early stage.
They did bring me in very early, and increasingly startups are looking to build data teams earlier than you’d see some other functions kick off within the company. There are lots of very young companies that have reached out and asked for advice about how to build the data science team from scratch, because they’re looking to do it straight away.
Adam: By doing it straight away, what doors are opened that might have been more difficult for you to pry open if you came in a year later?
Riley: When you think about the early days of the startup it’s really a fight for survival. Every minute that you spend on something is a very critical decision. Data is all about being the rudder on the ship, pointing the ship in the right direction, and making sure that what you’re doing is actually impactful. It’s checking your gut assumptions about what we think is the right thing to do and ensuring the assumptions are actually right.
It’s actually more important that early stage startups build a strong data culture early, because in many cases it can be the difference between death and success.
Be data-informed, Not data-driven
Adam: You’ve spoken elsewhere about the idea of being data-informed rather than data-driven. How do you strike that balance between getting the most out of the data available to you but appreciating that data doesn’t always give you the full picture?
Riley: It’s very important to not be overly dogmatic about anything you do and maintain perspective that takes into account the bigger picture. A lot of people say you only optimize what you can measure. And the truth is you only measure what you can log. There’s always this risk, particularly in a business like ours that’s primarily offline, that you will spend all of your time optimizing the online portion of the business and forget the actual experience that the guests and hosts of Airbnb. That’s what they think of after their trip, what did I do offline?
Another critical element for a data team is respecting the limits of data. You can go as deep as the things that you are able to measure. A good example of a powerful dynamic that has developed here is the relationship between our team and the more qualitative user research team.
It doesn’t work when the two teams have conflicting perspectives of the world. The user research team will interview a couple people and say, “Across these three people, this seems to be a common theme or problem.” Then you’ve got a data science team that can’t measure the things they’re talking about, and they say, “That doesn’t make any sense.” They’re totally at odds, and that’s terrible.
There’s a different dynamic, which we’ve discovered here, that is much more powerful, and it’s all about the hand-offs hypothesis. The data science team can identify an anomaly and pass that anomaly off to the user research team, who can go deeper than the data lets us. They can bring back a hypothesis in the form of a solution to the anomaly, which we can help put into production and test through a controlled experiment.
To give you a concrete example, we were looking at the conversion rates of our payments page and were cutting it by the country of origin of the person who’s trying to book. We saw that one country was pretty far off the rest. That was all we could say. We didn’t know what was happening, so we sent a group of user researchers to that country to talk to people. They found there was a standard method of payment people from that country preferred to use online, and it wasn’t something we offered. With that insight we were able to test the offering of that payment instrument, and all of a sudden the country took off.
That was not something we could have figured out from the data alone. It’s a good example of the respect a data science team should have for the limits of data and ensuring the company is very informed but not overly dogmatic.
Adam: Does that play into your idea that data is really the voice of the customer? At an experiential company such as Airbnb that concept has to be particularly important. How has it framed your approach?
Riley: That’s very deep in the DNA of our team and the company in general. Brian Chesky and Joe Gebbia, two of the three co-founders, talk a lot about how in the early days they would fly out to New York to meet with the first hosts of the Airbnb community and talk to them about what was happening, what worked for them about the experience, and what didn’t work. Then they’d come back to San Francisco and meet with Nathan Blecharczyk, the third co-founder, and try to iterate on solutions to those problems.
Being very close to the community and listening to them was a powerful feature of Airbnb’s early success. I view the data science team as carrying that culture forward as the company has scaled – trying to keep us very much in touch with the people who are using the product. It’s no longer possible to meet with every single person individually, but we do try to host meetups and stay connected to guests and hosts.
If you think of what data actually represents, it’s a decision that somebody makes. When somebody comes to book on Airbnb – say they perform a search for a place to stay in San Francisco – they’re presented with a set of results. They click on one listing, come back and click on another, and then ultimately book.
Probability of booking in a given neighborhood for Airbnb guests searching in San Francisco
That is a powerful signal for us about the decision they made and what worked for them. We can’t sit with every single person who’s trying to book on Airbnb and try to understand why they chose that listing, what it says about their preferences, etc. But we can log that information, and we can try to learn from it. Our role as data scientists is to keep the company in touch with those decisions and experiences that guests and hosts have.
The economics of “markets”
Adam: For a global company such as Airbnb, the differences between markets are such a huge challenge. How does your economics background shape your view of this?
Riley: It’s super important, because that was the first problem I worked on. When you’re building a two-sided marketplace, there’s a real cold-start problem. You have a product, but it doesn’t work until you have people using it. It’s a chicken and egg situation.
This really resonated with me, because when I was in grad school I focused on economic geography – how economic trends take place across space and how those different spaces or clusters influence one another. It’s also something I spent some time on while at a consulting firm before Airbnb. We were looking at the 2008 recession and trying to understand how it would play out across different cities and states around the country.
Economic trends and data shape how Airbnb approaches each of its markets.
When I came to Airbnb, it was a very natural extension for me to think about the world as the sum of a series of markets. What is happening in New York, and how can we learn from that for what we need to do in Paris? That’s really where we began. How do we get a strong base of supply in these cities, how do we drive demand to these cities, and then how do we match supply and demand appropriately?
A component of culture
Adam: Data information has become a core component of Airbnb’s company culture. What advice do you have for a startup that wants to replicate that?
Riley: There are a lot of things that really make a difference when building a strong data culture within a company. Number one is data scientists are only as impactful as the context they for the set of problems they’re meant to solve. This is a really important concept behind the structure of a data team.
When you look at lots of different companies, the structure of the data science teams tend to vary quite a bit. In some cases they’re very centralized and it’s this ivory tower of knowledge. In other cases they’re very embedded and very tactical. The choice that a business makes about that structure will have important implications for the culture of data in the company.
We’ve tried both, and like many other places that have gotten this right, we landed on a model that’s kind of a hybrid of the two. We have a data science team, which is where people’s careers unfold, but that team is broken into these sub-units that are embedded with engineers, designers, and project managers. They have lots of context into a problem, and their work is very applied toward a clear outcome.
When you have much more of the centralized unit of data scientists, the team can move around to the most pressing problems facing the business, but they don’t have as much context when they get there. The analysis of their work tends to be more surface level.
That’s one really important concept. Another one is there’s a long funnel of activity that has to take place before a data scientist can really do impactful work, and it’s important for startups to understand and respect that.
A lot of investment is required into the way data is logged and the infrastructure surrounding where it’s housed – the ETL pipelines for transcribing it from a raw log event into meaningful information. In the absence of onerous for all those things, you’ll find that data scientists want to work at the more analytical or machine-learning end of the spectrum. Generally they’re not well situated for the infrastructural stuff, which is more of an engineering problem.
But if a company doesn’t respect the need for investment on those fronts, the data scientists are really handicapped. That’s another piece of advice I tend to give startups. Make sure you’re investing in data.
Adam: If you do find yourself as a data scientist at a young company and you are running into those walls or feeling handicapped, how can you demonstrate the value of analytics across the company? Is that something you ran into earlier in your career?
Riley: It’s all about identifying opportunities and doing whatever you can to highlight the solutions to those opportunities with data. Where those opportunities exist will vary from company to company. But there is a certain amount of evangelism that’s required of this way of thinking. In many cases, early-stage startups have a lot to learn about everything surrounding a business. This is just one of those areas.
A big part of the impact of a data science team is communication. When you’re working with a group that is meant to take action based on a finding from a data scientist, they don’t really worry about the statistical voodoo behind the work you’ve done. When data scientists come in and show the R-squared on their model and the coefficient on the features, it goes right past everyone in the room. They have no idea what you’re talking about. That’s not the point. They assume your work is statistically valid; what they want to know is, what is the story behind this? How should we interpret or understand what you’re telling us?
From that perspective something that we did early on that made a big difference is we placed equal weight on a data scientist’s ability to communicate their work as the technical rigor behind it. So that when they came in we could ensure they would be very impactful and help teams understand why this way of thinking makes sense, why we use experiments to roll out products, and how we interpret those experiments.
Data accessibility for all
Adam: What challenges have you faced with data accessibility at a company that’s scaled so rapidly?
Riley: That’s a big one. In the same way we help the company stay connected to guests and hosts, we’ve helped scale the quality of products that we build. As a company grows you also have to think internally about how your work scales across the base of employees that exist there.
When Airbnb was 10 people it was pretty easy for me to sit at the table and remind people of x, y, or z. When Airbnb reached 2,000 people that was a really different problem. There’s no way for a data scientist team to scale linearly within a hyper-growth business. It just doesn’t work. We spend a lot of time trying to look for ways to create leverage for the team and scale the work of every individual within the team. In many cases that means democratizing our knowledge of or access to data. We’ve spent a lot of time building tools and designing training for the company in how to access data and how to understand it.
Riley’s model for scaling data science across the organization at Airbnb
We also look for ways to generalize solutions to problems we face. In the early days it was very easy for us to stay in sync with each other, because there were only a couple data scientists. We all knew what we were working on, and there was a lot of tribal knowledge built in the team. But as the company and the team scaled, that started to break down.
We used to joke that there was as much seasonality to analysis as there was to the business. We’d answer the same questions year after year, which is super frustrating. Every time you’d get back to a given question, it’d be like “How did I do this last year?” You’d dig around in Git for code and look in your email for the keynote presentation. It’s a nightmare.
This gets back to the question of democratization or generalizing your work. We invested a couple months into building a tool that we now call the knowledge repo, which is basically like a Git repository where people check in IPython Notebooks. It’s a little bit fancier than that, but in a nutshell that’s what it boils down to. That has really helped the team scale its work and learn from each other.
From my perspective as head of a data science team, you have to look as much for opportunities for leverage within the team as opportunities for impact within the company.
Adam: Product managers, designers, UX folks – they’re all fighting for accessibility to data. What’s your advice for making sure they’re getting the most out of the data available to them?
Riley: Many of the designers and PMs at Airbnb, when they think about the world a couple years ago, they would’ve been just as frustrated as data scientists with the difficulty of accessing data.
Data scientists would find themselves being asked repeatedly to answer really basic questions or for really basic information. How many listings do we have in San Francisco? How many people searched yesterday? That is not how anybody wants to spend their time. No data scientist wants to be writing those queries day in and day out. And there’s no PM that wants to have to ask those things day in and day out.
It speaks to the need for investment in infrastructure and tools surrounding data so that the company is as empowered as possible and the team is able to focus on the highest impact work.
Adam: How does an early-stage company that might be based in a living room but doesn’t have you sitting in it begin introducing this line of thinking?
Riley: They start by bringing in someone who can spearhead this function of the company. I think the role of a head of data science or a leader in analytics is very much about thought leadership and teaching people how to think about things. It’s the role of the people within that organization to expand your knowledge of the ecosystem of your business, alongside partnering with engineers to build data products.
In each business case it’s slightly different, but it will be very obvious to the head of this function where those gaps lie, and that person should take responsibility for addressing them in doing whatever they can to democratize their team’s understanding of what’s going on and help create leverage for the people on their team.
Solutions at scale
Adam: Obviously you’re on the opposite end of the spectrum now. What’s the biggest problem that you’ve been able to overcome and solve in your time at Airbnb?
Riley: When you’re in this role for six years it’s different from one year to the next; it’s always something new. In the early days my focus was very much on tactics, and there were some really interesting technical problems that we worked through to grow Airbnb. As time has passed, and as the company and the team has scaled, the problems that I find myself solving are more organizational and strategic in that sense. Everything within a company boils down to the people behind the work. If those people are set up the right way for success then things tend to work out pretty well.
I find the most leverage from my work these days is really empowering the team of data scientists that I work with and ensuring they’re set up for success.
Adam: How large is your team now?
Riley: Roughly 75. We’re mostly focused here in San Francisco.
Adam: Where can your team continue to grow and explore in the field?
Riley: There’s a lot of things that I’m really excited about right now. We’re building out a data visualization team, which is pretty cool. As we have gotten to a point where we have lots of data scientists, information, and technicality within the team, I still find us trying to answer the question of what is going on and how do we frame it in a way that is digestible to a fast-growing business? Data visualization is so critical in that regard. We’re building a team that can serve as thought leaders within the data science organization and think about how to frame problems different ways and how to communicate a compelling and consistent narrative across different projects as well as across the business as a whole.
Of course there’s also machine learning. There are a lot of people that are coming out of PhD programs with a lot of interest in this area, but there are still many different ways of approaching the concept. Here at Airbnb we’re thinking more about how to standardize the infrastructure behind this, and again, to democratize it so everyone is able to partake.
A third area I’m very interested in is thinking more about how you log offline activities. What is the right way to get better signal about what people are doing with Airbnb when they’re not just online? I think that is the most important thing for us as a business to understand.
Adam: It seems like quite a large gap to fill. Riley, this was great. Thanks so much for joining us today.
Riley: Thank you.