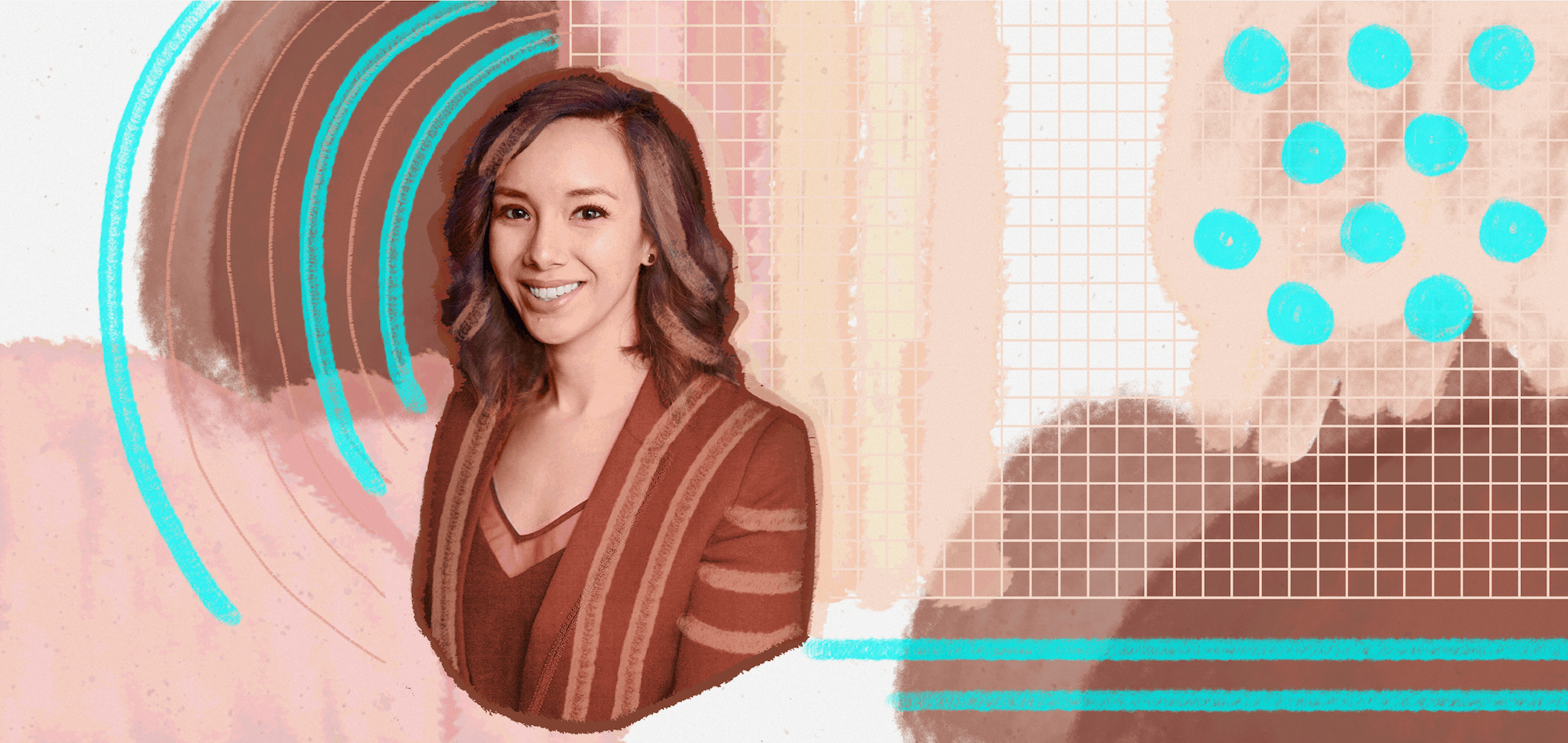
Optimizely’s Claire Vo on the power of product experimentation
If you ask Claire Vo to describe product managers with one adjective, it's greedy.
It’s a term that Claire, VP of Product Management at Optimizely, uses endearingly. After all, PMs want more adoption for their product and more usage of their features, and it’s that greed that drives them to continuously experiment with new and better solutions.
As an organization scales, managing experiments – and democratizing their learnings – quickly becomes complex. Solving that specific problem is what led Claire to build Experiment Engine, which Optimizely acquired in 2017. Prior to that, Claire led product management and user experience teams at several eCommerce and tech companies, including Electronic Arts and uShip.
I hosted Claire on our podcast to learn how her product team selects and prioritizes its experiments, ways to make sure results are shared and accessible company-wide, and how to source more experiment ideas from outside your product team. If you enjoy the conversation, check out more episodes of our podcast. You can subscribe on iTunes, stream on Spotify or grab the RSS feed in your player of choice.
What follows is a lightly edited transcript of our conversation.
Adam Risman: Claire, welcome to Inside Intercom. There are a lot of very different paths into product management. What was yours?
Claire Vo: I have one of those highly lucrative liberal arts degrees, so I got a non-traditional academic start in product management. My first job was at uShip, in Austin, Texas. It was a classic startup PM role where I was the employee number 13. I gravitated towards product because you really got to do everything at that stage. You’re building marketing, you’re building messaging, but you’re also building experiences. Ultimately I’d go on to run several product teams including one at Electronic Arts. At that company, I really decided I was ready for entrepreneurship, and I had this idea about how to make experimentation at scale easier.
I then founded Experiment Engine, and I ran that company for about three years, until it was acquired by Optimizely. Today I’m the VP of Product, and I help run all our product strategy, development and design teams.
Adam: What’s the size of your product team, and how is it structured?
Claire: We have about 10 people on the product team, and then a handful of product designers. We have a product team focused in three major areas. We have a team of PMs focused on our experimentation products, which include our web experimentation, web personalization and full-stack server-side experimentation products. We have a second set of PMs focused on our data products, which include our events pipeline, data processing, statistics and all of our reporting around experimentation programs. The third group of PMs is really focused on the product that I built at Experiment Engine, which was ultimately integrated into the product suite at Optimizely and is now called Program Management. It includes all of our workflow and collaboration tools for teams running experimentation at scale.
Creating a culture around experimentation
Adam: When it comes to experimentation in product teams, what does a healthy culture of experimentation look like to you?
Claire: In terms of a culture of experimentation, the most important thing is approaching everything with hypothesis thinking. There are no genius product managers, right? There’s no one who just absolutely knows how a customer is going to react. There are very smart and strategic, and organized, and thoughtful and data-driven product managers. But the best product managers really approach things with the mindset of, this is a hypothesis that I have, this is how I’m going to measure whether or not I’m right, and I’m going to cycle through that quickly to get to the answer for the business and for the customers.
Approach everything with hypothesis thinking
Adam: In a lot of companies, hypothesis thinking gets siloed within product management. Obviously, it’s core to the job, but how do you spread that to other disciplines? What role should, for example, engineering, design or marketing play?
Claire: The company that I built, Experiment Engine, was really founded on the concept that enterprise-wide experimentation was the only way you could really scale a program. One of the key problems we tried to solve was how do you break down silos between departments, and truly build an end to end culture of experimentation? Not just in product engineering and design, but in marketing, customer success, sales, the executive team, etc. We built a SaaS platform for managing your experimentation, capturing ideas, prioritizing ideas, collaborating on bringing those to life and then capturing the results from those experiments. We use that product today to solicit ideas from across the organization.
We also have a weekly meeting that we call Experiment Review, where people who have submitted those ideas can actually come present them to a cross-functional group of engineers, designers and customer success people. They’ll collectively decide whether it’s a good idea. If it is, we should help resource running the experiment. It’s a great way to bring in experimentation from across the organization.
Setting up an Optimizely experiment
Adam: Can you put us in that room? What do those meetings look like?
Claire: We spend the first half of the meeting inspecting a couple of specific experiments. These are either ideas that we want to get promoted through the approval flow and into implementation, or experiments that have already run. For the latter, we are looking at the results and as a group trying to understand the data.
When someone is presenting their experiment for prioritization, we’re really inspecting whether this reflects a customer problem. What is the basis of your hypothesis? Do you have data? Do you have a qualitative or quantitative understanding from our customers that makes this a valid hypothesis? How are you going to measure this? Is this something that we can actually measure, given how our product is instrumented? And is this something we can potentially get to statistical significance with or not in an experiment in a reasonable amount of time? We’re really looking at the structure of a hypothesis, how you’ll measure it, and if it’s actually possible. Sometimes we’ll get into the design of it and have debates on the best way to frame the experiment.
Most experiments get through, although it sometimes takes a couple cycles of refinement. This process helps us train all parts of the organization on how to frame a good experiment, how to build a good hypothesis, and just by being in that room, somebody’s idea gets better a week down the road. It’s my favorite meeting of the week.
Adam: Is there a prioritization framework that your team leans on when approving these experiments?
Claire: Prioritization was one of the big problems that Experiment Engine was trying to solve, so we brought our prioritization framework into Optimizely Program Management. We have a model called the PILL Framework
- P is for potential: the potential of the test to increase whatever your metric is; how likely you think it is to win?
- I is for impact: If the test does win, how high of an impact does it have on the business? For example, if you’re in eCommerce, something that’s going to increase revenue per visitor is much higher impact than capturing an email address.
- The first L is for level of effort: This is typically engineering level of effort, but we think about it as an end to end level of effort for implementing the experiment.
- The last L, which is my favorite, is love: how much you just like the idea? I’ve found that a lot of people will skew those more quantitative metrics based on how they feel. And what they’re really trying to convey is, this is high impact, it’s high potential, it’s pretty easy to do, but I just hate this idea. We wanted to give a place for people to air their grievances.
Spreading and documenting results for greater impact
Adam: What are some of the things your team does to help democratize the learnings from your experiments so everyone knows what’s going on, and what pieces of the product or funnel might be changing? There’s got to be some sort of historical record, right?
Claire: That was my question when I started Experiment Engine. There has to be some sort of historical record. I visited a company that ran about 250 experiments per year, pretty high volume, and put tons of money into their experimentation program. I went in and was going to help them scale this up, and I asked, “Can you give me an overview of what experiments have won and lost, and the themes of your experimentation program, what it looks like, etc?” They said, “Oh, well, Bob knows.”
What if Bob gets hit by a bus? I was flabbergasted, and I said, “Okay, this is a solvable problem.” Part of what is now Optimizely Program Management is this searchable, filterable archive of experiment learnings. It’s not enough to have the results page, and Optimizely has this lovely statistically rigid, rigorous results page that will tell you how much confidence we have in the results and what the specific metrics are.
It’s not enough to have the results page
But, if you’re coming into that results page a year later with no context, you probably don’t have any idea what happened. So one of the capabilities we added was just the ability for someone to annotate the results. Let’s say, as a business, we decided this variation was the winner and this one was the loser. Our documented analysis is put in a repository for you to search. So if I want to go to our marketing team now and say, “Show me all the headline tests that won,” I can see all the headline tests that won. Or, if I want to go to my product team and say, “Show me all the results page experiments that were inconclusive and didn’t move the needle, so we don’t repeat it,” It’s now all accessible in this repository.
The other thing that we do, particularly for engineering, is we write all the experiments that we run in a quarter on a big wall, and it’s one of the key things that we track. We set a goal at the beginning of the quarter and then we fill it in, so everybody knows what’s being experimented, where we’re experimenting, and where the high priority stuff is.
Adam: Do you have actual goals for your individual product managers for experimentation?
Claire: I do. This was one of the things that I thought was really important to bring into Optimizely, and something I’ve seen be really effective in driving velocity in experimentation programs. My whole philosophy is you can’t move it if you don’t measure it. You can say you want to build a culture of experimentation, but what does that actually mean? The simplest thing you can say is, “I want to run X experiments in this time period.” At the beginning of our fiscal year, Q1, we set a very baseline experiment goal, which was to run six product experiments that quarter. We’re B2B enterprise, so we don’t have super-high volume like a consumer site would, and an experiment every other week seemed perfectly reasonable.
We hit that goal, so for Q2 we said we were going to run 18 experiments. That was inclusive of engineering experiments, which are typically feature roll-outs, and what we found is that about 15 of those experiments were actually run by engineering. My product managers slacked a little bit. So I’ve been giving them a hard time because they’ve been helping their engineering partners run experiments but they themselves have not been doing as much product experimentation as I wanted. So for Q3, we’re doing 10 product experiments, 15 engineering experiments, and my product managers sign up for a subset of that – it’s basically one and a half per product manager. We’ll measure it in separate lanes on our wall and we’ll make sure it happens. It’s a simplistic way to view it, but it’s effective, particularly when you’re just getting started with your experimentation program.
Pinpointing your primary metric
Adam: What are your principles when it comes to metrics? Can experiment focus on multiple data points, or do you prefer to see one primary metric?
Claire: I like to see a primary metric. We have a formal experiment brief – fields and information you have to fill out when you’re proposing a hypothesis to be run as an experiment. One of them is, what’s your primary metric? All things being equal, if this metric moves will you make a decision on this experiment? I like to define that because it really clarifies the customer problem that you’re trying to solve.
It’s useful to have supporting metrics to provide you context, but it’s good to know from a hypothesis framework that if we do X, users will do Y as measured by Z, and that Z can’t be as measured by every metric under the sun. If you get your hypothesis framed in a really simple way and get clear on your primary metric, that’s a good starting place.
Adam: Does that help you for scoping that experiment as well?
Claire: It does. It helps for scoping the experiment, it also helps for scoping the amount of time an experiment would take to run. If we look at things like more downstream metrics that don’t happen very frequently or happen at low volume, those aren’t particularly good primary metrics because they take so long to get to statistical significance.
Measuring results in Optimizely
Adam: What’s an example of one of those?
Claire: In retail, a good example is returns. If you’re doing an eCommerce experiment, and you’re ultimately tracking not only do they purchase it and does it arrive to their house, but that it ultimately gets returned, that’s just going to take a really long time to get to statistical significance. The difference between the experiment point and the point of the metric you are measuring is very far apart. But if you do something like conversion rate or average order value, that’s much closer. I do think those further along metrics are really important, and in some high-volume industries or high-volume companies you can actually experiment with those, and that’s what we see very high maturity customers do. As a B2B enterprise product, however, we don’t have the ability to wait six months for an experiment to reach statistical significance on some far-fetched metric.
Playing into greed and fear
Adam: Does the sales team ever put you in touch with product teams at other companies who are struggling with experimentation? And if so, where do you see them tripping up?
Claire: We talk to product teams all the time, and we get a variety of questions from them. A common one is, what kind of things should my product team be experimenting on? We also hear, “My product team already knows what to build so don’t tell me to experiment.” That’s sort of a cultural shift that you have to make, to challenge those assumptions.
The most successful companies in the world
have built experimentation as a core competency.
The more interesting piece we’re asked about is how do we get engineering on board with this being a core competency of a sophisticated, mature engineering organization. How do we tell the story that experimentation, feature flagging and gradual rollouts are part of a continuous deployment story that ultimately results in faster engineering velocity and better quality, even though it requires a little bit more work? Product people tend to be our allies in that conversation, we just need to bring the engineers along.
Adam: We have a saying here that shipping is our heartbeat, so we’re big believers in that concept as well. Is there anything that you’ve really seen to help shift that mindset?
Claire: There are a couple things. One, you point to engineering organizations that people admire, and you say this is just business as usual for them. The biggest and most successful companies in the world have built experimentation as a core competency.
The second thing that you can do is really play to the value that you’re driving for each of these organizations with experimentation. On the product side there are two reasons you experiment: fear or greed, probably the two reasons why you do anything in life. Product managers are greedy. Product managers want more adoption for their product, they want more usage of their features, and they do greed-based experiments. “If I do this, my customer will use this product feature. If I do that, I’ll get to acquire more users.” So you really speak to the greediest cases with product managers.
Engineers you tend to speak to the fear use case. “How do you know that this isn’t gonna break something? How do you know that this won’t have some small bug that you didn’t catch that then hits your most strategic customer? How do you know you’re not going to spend X dev hours rolling back a big release you did because it didn’t work for customers?” Frame the product experimentation story around how you can get stuff as a product manager and be greedy. The engineering side of the story is really about the quality of velocity and risk mitigation. You want your deployment mechanism separate from your delivery mechanism to customers so that you have more granular control over it in case things go sideways.
The painted door test
Adam: The voice of the customer has to play a role here as well. What are the types of ways you advocate for your product managers to stay close with your customers?
Claire: I don’t know if this is particularly unique to enterprise, but my product managers spend a lot of time in-person, on the phone, in video conferences, and email communication with our customers. If I ask in any given month which customers my PMs have met with, I get a list back of like 50 different ones. We spend a lot of time with customers really understanding their use cases. It’s a luxury that we have as an enterprise company, because we don’t have millions of users of our product and we can really go to some of our most strategic or most mature customers and have long, deep conversations with them.
The real power in product experimentation lies when you can embed experimentation deep in your code.
The other thing that we do is we use experimentation to drive research engagement with our team. So often when we’re planning a new feature release or we have an idea for a new feature, but we’re not sure what customers think of it or if we’ve gotten it quite right, we’ll do what we call a Painted Door Test. This is essentially creating a live component that indicates that a feature is available when it’s not. Then, when a user clicks it we say, “It’s awesome that you’re interested in this thing. Would you like to talk to the product manager about your needs?” We’ve been able to solicit really great conversations out of modules like that, which then sometimes dramatically change the course of direction that we go with a product. It’s been really fun to use Optimizely as a way to capture qualitative feedback from customers about what they want from our feature set.
Adam: Speaking of Optimizely, what’s next for you and your team, and where can we keep up with the latest happenings there?
Claire: We’re really focused on this product experimentation use case and making our server-side experimentation tools even more powerful. What’s interesting about our product team is we use our client-side web experimentation product just as much as our server-side product. We use it to do these promotions and point people to features and do Painted Door Tests. But the real power in product experimentation lies when you can embed experimentation deep in your code, and so my product managers think deeply about how to service other product managers and product leaders to drive value for their customers.
We also want to provide leadership and insights on how to do this. We have a product experimentation blog, where we talk about best practices, common pitfalls, big mistakes, funny things you hear in experiment review, that just give other teams some insight into how to actually do this on the product side.
Adam: We’ll be sure to check out those resources. Thanks for joining us, Claire.