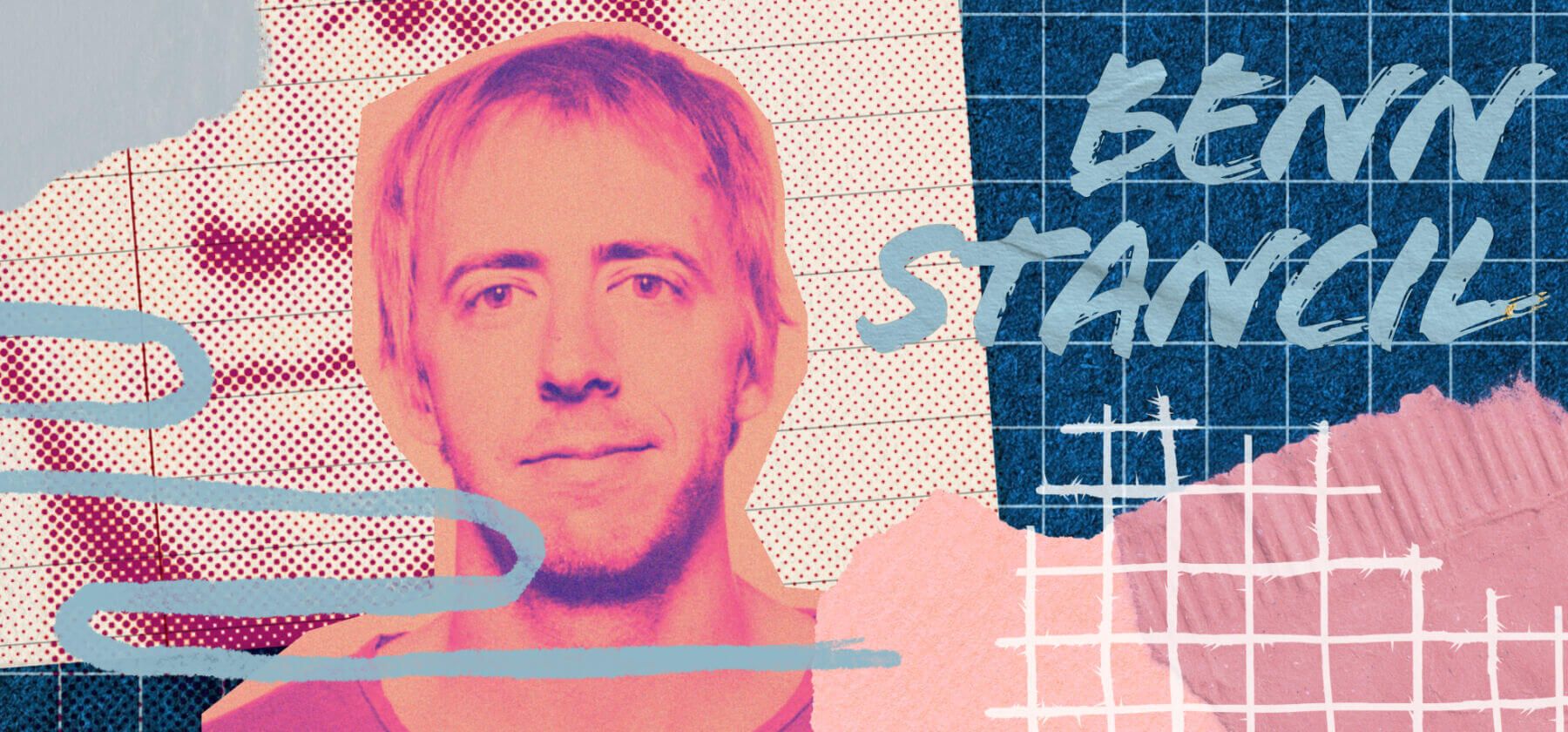
Benn Stancil, founder of Mode, on how data science can help us make better decisions
Data analysis is not about spewing fancy dashboards – it’s a long, iterative, collaborative process that helps you to better understand what the customer wants, and how to get there.
Or rather, it should be. That stereotypical image of a room teeming with monitors and elaborate dashboards where all the decisions are made isn’t real, and it’s high time we move away from it. According to Benn Stancil, co-founder and Chief Analytics Officer behind Mode, a platform designed to help data analysts and data scientists analyze, visualize, and share data, a lot of their actual day-to-day work involves sitting with product managers and business leaders, tinkering with various things, trying to solve a business problem together.
The fact of the matter is that data science, as a field, is still relatively new. And so, many business leaders find themselves not quite sure how to start, how to leverage it, or what to do when all the dashboards and tools seem to be pointing at different solutions. But as Benn points out, the future of data analysis isn’t an architecture diagram or business leaders looking at dashboards – it’s building an experience, and a very exciting one at that.
This week, we had the pleasure of chatting with Benn about the modern data stack and how data can – and more importantly, should – be used to drive growth.
If you’re short on time, here are a few quick takeaways:
- Although data science can’t predict the future, it can definitely help you focus resources, troubleshoot issues, and even unlock new markets.
- Any insight from data analysis will only ever be as good as the data itself. Make sure your data is accurate before you draw any conclusions about it.
- Unless you’re looking at the data to understand why your customers are using your products, avoid making decisions based on your expectations of their motivations.
- As we see more standardization over best practices for handling data, it will no longer be necessary to design the process from scratch, but rather build on top and adapt them to each use case.
- Looking at endless rows and columns can be daunting even for a seasoned data analyst. When in doubt, start poking around with some basic product assumptions and go from there.
If you enjoy our discussion, check out more episodes of our podcast. You can follow on iTunes, Spotify or grab the RSS feed in your player of choice. What follows is a lightly edited transcript of the episode.
Getting into analytics
Liam Geraghty: Benn, thank you so much for joining us today.
Benn Stancil: Great to be here.
Liam: First of all, could you tell us a little bit about your background, what you do and how you got to this point?
Benn: I basically started my career in a totally different world. I started in the policy world as an economics researcher at a think tank. I spent a couple years there, really liked the work, and liked the idea of basically trying to solve problems with data. My job was to look at what was happening in the world economy – it was like 2010 at the time, and so everything was kind of falling apart – and then look at data about it and try to come up with recommendations about what policymakers should do.
It was really interesting conceptually, but if you make recommendations about what the Fed should do as some junior person at a think tank, nobody’s really paying attention to you. I like the idea of doing that kind of work, but I wanted to do it in a way where it was more directly related to the problems people were trying to solve, where you could actually see if your ideas or recommendations worked out. That’s how I ended up moving from that job into an analytics job at a tech company in San Francisco. That was my first job in tech. I worked there for a couple years as an analyst or data scientist. I was the type of person whose job is to work with marketers, product managers, or operations folks to help them make decisions about which products to ship, which audiences to target, or if the business is performing well in certain areas and not well in others. It was similar to what I was doing in DC but applied to a very different domain.
“The journey of a company of three people working out of someone’s living room into something that’s hundreds of people is one where you constantly are encountering new things”
From there, I met some folks, started realizing the needs of folks like me and what kind of tools we wanted, what kind of tools weren’t there yet, and ended up creating Mode based on that vision.
Liam: Was it challenging to get to this point with Mode in the startup journey?
Benn: For sure. Every startup is going to be a challenge, and Mode is no different. Mode has had plenty of ups and downs. On the one hand, it’s been very challenging. Every day you work on different problems, stuff that you’ve never really anticipated before. As I’m sure you’re well aware, the journey of a company of three people working out of someone’s living room into something that’s hundreds of people is one where you constantly are encountering new things, where the problems you have on month one are different than problems you have on month two.
Every day, you’re having to learn some new thing that you’ve never thought of having to solve before. It’s not like, “I’m going to get really good at this particular thing.” Just as you get good at it, you’re onto the next one. In that sense, it’s been very challenging. However, we’ve had a great team. There are great people who supported the company, whether or not they’re the employees, whether or not they’re people who were early customers, whether or not they’re just friends and family, and the types of folks who put up with you as you’re going along with it. It’s been hard work, but it has been a very good experience, having the support of so many folks to do it. I think folks in Silicon Valley are particularly privileged to have an entire ecosystem dedicated to helping them and their company succeed. If you were to do this in other places, I think it would be much harder.
What data can and can’t do
Liam: Data science is what it’s all about for businesses looking to improve their decision-making by using the information they’re already collecting in their apps and other systems. This is where your company Mode comes in. Can you tell us a bit about the platform you’ve built and how it helps data scientists?
Benn: For sure. Companies use data in a lot of different ways. The easy way to think about it is thinking about what you see in caricatures on movies and stuff, like rooms full of dashboards and charts where people are making decisions. That obviously doesn’t happen. People do have dashboards and we’re all checking how companies are performing and that kind of stuff. But a lot of the actual day-to-day work of using data effectively comes from a data expert sitting with a business expert trying to solve a problem together and answer the questions they have to help them make some decisions.
“The product manager is not going to look at a screen and be like, ‘Aha, this is exactly what we need’”
Imagine you are a product manager wanting to decide which product to build. You may want to understand how people use the current features that you’re thinking about changing. Do different types of people use it in different ways? Are there things they’re trying to do but are struggling with? Can they accomplish the goals they have? All of those things require these very particular questions, and it’s not a process that you would answer by looking at a dashboard. The product manager is not going to look at a screen and be like, “Aha, this is exactly what we need.” It’s this long, iterative process of understanding more and more what their customers want, then coming up with a decision and saying, “Hey, I think we should build this. Let’s do some analysis to figure out if we think that’s the right feature to build.”
Mode is really about enabling people to do that. It’s about enabling that analyst to do that sort of work so that they can very quickly and easily help answer questions for other folks. And then, how they distribute that and make sure it’s collaborative with those people as well – an analyst can’t just go into a hole and answer these questions, they have to work alongside the product manager to do it. That’s what we really want to enable. And once you have those kind of answers, can you turn them into something reusable? Can you make them so they’re easy to return to and auditable and all that kind of stuff? At the core of it, it’s about helping people who need to understand something about their business and answer questions by enabling them to work more fluidly with analysts that are often the ones driving those answers.
Liam: What’s a data story or example that had a happy outcome and one that didn’t so much? Just for people like me who are dipping their toe into the pool of data, for example.
Benn: A couple of happy outcomes. There was this company that was basically serving videos around to a bunch of their customers – their product is a global video platform. They were trying to figure out how to make it better for people around the world, and they realized that some countries tended to perform very well and some countries didn’t, and they weren’t exactly sure why. They were like, “Oh, we’re doing great in this country and doing great in this country, but not doing so great in these others.
“[Our research] opened up new markets for this product without them having to go through the other kinds of experimentation they were originally planning”
After doing a bunch of analysis and digging into it, they realized the videos were too slow in certain countries because of where their data centers or AWS environments were located. They thought maybe it had been something cultural, something about internationalization, that maybe they had done something wrong there. Maybe it was something about the product that just didn’t resonate with particular audiences or that was adopted by different types of people with different use cases in different countries. They had a bunch of different things they could have tried to solve, where it’s like, “Maybe we have to invest a whole bunch of internationalization, maybe we have to invest in different features if we want to sell to these types of audiences because they think about the problems differently.” Again, it was a video platform, so maybe they had to invest in different content.
But it ended up being something simpler. The app was too slow in these countries, people got frustrated because the videos would buffer too much, and it just wasn’t a good experience. They could solve that by saying, “Hey, let’s put our product in more data centers in AWS,” or in whatever hosting product they were using. It’s not a trivial solution, but it’s not a hard solution comparing to having to figure out how to create entirely new content for different international audiences. It opened up new markets for this product without them having to go through the other kinds of experimentation they were originally planning when they realized that they were successful in some countries and not in others.
Liam: Absolutely. That’s a great example.
Benn: In terms of the negative data stories, I think there are a couple examples. There are certainly cases where people will try to make data-driven decisions and end up making something that doesn’t work out. Part of that is the nature of what data is – it’s telling you something more or less probabilistically. If you ask, “Should I bet on me rolling a die and me getting between one and five or a six?” I could tell you, “The right thing you should do is bet on a one through five, your odds are much higher for that.” That’s the right data-driven analysis, but sometimes you may lose. If you’re building a product or making a decision for a business that you can only make once, you can’t go back and say, “Actually, let’s roll it again and keep rolling until you get a one through five.” Maybe we bet on a one through five and we rolled a six. That’s how it goes.
“That’s part of the game of trying to be more data-oriented as a product and a business – you’re not always going to get it exactly right”
There are a lot of examples of that sort of thing where data’s not perfect. Data is not going to tell you the future. It can give you some sense of what might happen, but there are a lot of stories about people making decisions, probably doing the analysis really well, making what is a sound decision prior to it being made, and then the result just doesn’t pan out. One of the things we talk to a lot of folks about is being okay with that. That’s part of the game of trying to be more data-oriented as a product and a business – you’re not always going to get it exactly right. You just can’t lose faith in what data is by expecting it to always tell you exactly what the future will be.
Garbage in, garbage out
Liam: Well, off the back of that, how important is it to consider the journey that data follows to reach the dashboard at the end?
Benn: There’s definitely a “garbage in, garbage out” type of process to all this, and I think that’s pretty well understood. Most people understand that data quality matters. Say we’re trying to make decisions about our sales pipeline and how well different customers are moving through a sales pipeline and which stages of the sales funnel perform well and which ones don’t, you may come to the conclusion that deals always get hung up in security review when we talk to folks on IT and security about whether or not our product meets their standards, and so, we need to focus a lot more on that. If our conclusion is based on data that is manually entered by a sales team, it may just be that that’s the part of the process where the sales team is sloppier about entering that data in Salesforce, and therefore, that conclusion isn’t a reflection of reality. You’ve got to do all the work upfront to make sure your data is actually accurate before you can draw any real conclusions about it.
“It’s not necessarily that they’re fudging the numbers or that they’re using bad data – it’s just that when you go looking for something interesting, you’re much more inclined to find it”
There’s another way in which I think there’s a similar dynamic around this “garbage in, garbage out.” You can do a bunch of different analyses, and say you look at some problem from 10 different perspectives, the way you look at that will matter. There will be some perspective that appears a particular way, and that analysis, by itself, may be sound. But unless you tell people, “Hey, we tried 10 things, nine of them suggested one thing, but this 10th one suggested something else,” and then the 10th thing that suggested the thing that’s the outlier appears to be very positive…
You hear a lot about this in the academic p-hacking type of studies where people are basically writing academic papers, and there’s this question of, “Are they looking for results?” The answer is, of course, they are. Academics are paid to do that. They want to find something interesting. It’s not necessarily that they’re fudging the numbers or that they’re using bad data – it’s just that when you go looking for something interesting, you’re much more inclined to find it, even if that interesting thing isn’t necessarily real.
Analysts can suffer from the same tendency. Our job is to find something interesting. We want to be the one that stands up at the big meeting and says, “Check out this chart that reveals this really dramatic thing.” If you’re always looking for those things, again, nine times out of 10, you don’t find it. But that 10th time, when you do, it’s important to say, “Hey, we tried a bunch of other things first.” That helps understand whether or not this thing you’re thinking you’re finding is real or not.
Liam: For startups out there, how can data be used to drive growth?
Benn: A bunch of ways. Most people use it like growth hacking, which people pejoratively referred to as a new way to call marketing, but you can use it very much in that kind of way. You can use it to better understand your customers, to know who to talk to. A lot of growth is just finding the right people and giving them the right message at the right time, at least from the top of the marketing funnel side. Data can be really important in that. How can you understand, from who’s coming in your funnel, what they say and how far they get? Who are those people, and how do you reach more that look like that?
“A lot of growth is just finding the right people and giving them the right message at the right time, at least from the top of the marketing funnel side. Data can be really important in that.”
The more important levers, though, are often around understanding what your customers are trying to do. People will often build products and expect them to be used in a particular way or expect people to like them for one reason or another, and people don’t always like it for those reasons. There are a lot of features that we’ve built at Mode or that we’ve heard from our customers where they’re like, “Oh yeah, this is going to be a great thing for X.” And it turns out that that’s not really why people like it, but they might like it for Y. Unless you’re looking at the data to understand the behaviors of what people are doing and trying to understand what motivates them to do those things, you’ll often think, “Oh, great, we launched a feature that was really good for this, and that’s why people are using it. So, we’re going to really lean into that.” But it may be that you’re actually playing against the way people are trying to use the product, and you should instead be saying like, “Hey, they’re using it for Y. Let’s really build ways it to enable them to do Y thing on it.”
“It’s about finding the actual product-market fit rather than the one you assume you have”
As an example, there was this company that was kind of a chat product essentially for work. It was a collaborative, kind of a Slack-type product that was mobile-focused. They originally thought it’d be a great way for frontline workers to communicate. It would be a great way for people who don’t have email addresses to communicate. If you’re a cashier at 7-11, you may not have a 7-11 email address, but we need a way to be able to have corporate talk to those folks. This was an app for that. They got some adoption, and they thought it was because of these people who didn’t have email addresses, but after looking at a bunch of the data, they realized it was actually people who had to be in and out of the office a bunch. The most useful thing wasn’t that they didn’t have an email address – it was that they were away from their computer a lot. They were essentially using it as a WhatsApp type of replacement for corporate chat.
So, had they thought it was about people without an email address, they might have built a bunch of features where you sign up with your phone number, where you do a bunch of things that make it possible to make this a thing where you don’t have a desktop computer. But what people actually wanted was this experience where they could use a computer sometimes, and then walk away from it and have a really great mobile experience while they’re on the road, or they’re making a delivery or whatever. That creates a very different path for that product and company, but they would never understand that unless they were looking at the data to understand the people who were actually using it and what they were trying to do with it. It’s about finding the actual product-market fit rather than the one you assume you have.
New tools, same problems
Liam: What does the modern data stack look like right now?
Benn: The modern data stack is the catchall term for the new set of data tools that are being developed on the backs of data products moving to the cloud. The core of this is the data warehouse. Before 2010, any company that wanted a data warehouse, which is where you store all of your data, would typically have to go buy something, a physical machine or physical software, like on a CD, that they would then run on a machine they own. We would have a server room in the back that is our database, and we would be running some Oracle database on it, and all of that. Basically, with Redshift, which was one of the first cloud data warehouses, people started moving all that to the cloud. Instead of me having to buy a machine or software, I can just go to AWS, say “Spin up a Redshift database for me,” I can do that from my browser in 15 minutes, and I don’t have to actually have any hardware to do it. A bunch of tools have been built on that idea of saying, “What if we did all of our data work in the cloud and didn’t have to have all this software that we ran ourselves?” The modern data stack is kind of around that idea of what it looks like for data to be moving to the cloud. There are a bunch of ways you can define it. But generally, it has been building these very horizontal products that will just ingest data from third-party apps.
“We’ve had these dramatic improvements in the quality of the technology, but the core problems that we’re trying to solve are often stubbornly persistent”
Say that you have data in Salesforce or Zendesk or Marketo or Stripe, products that will just ingest that data into your warehouse. You’ve got products that will transform it and model it in your warehouse – say you got a bunch of messy data that you want to turn into clean data, so you’ve got products that will manage that. The warehouse itself will do storage. There are analytics tools, like Mode, that sit on top of it for dashboards, for analysis, for being able to share and distribute that work. And then, there are a bunch of other tools that get built to support those. Now that you have five or six tools running your data tooling, you want to be able to monitor all of them. Is everything working right? Are the pipelines from point A to point B up and running and performing as I expect? If I want to make a change to one, how do I sort of orchestrate that change to the system?
It’s becoming this much more complex ecosystem, but it’s all built on this core flow of some way to ingest data in a warehouse, some way to transform it in a warehouse, some way to build a data asset on top of the data in the warehouse, and then some way to ship it off to whoever it is that actually needs to see it.
Liam: It can be so hard to get everyone to agree on the numbers when you have different dashboards and tools telling you different things. Is that a challenge?
Benn: Yeah. This is one of the paradoxes of the current state of the modern data stack. We’ve now spent close to 10 years building these products, and they’re revolutionary, in a lot of senses. They do things dramatically better and dramatically faster than they did 10 years ago. About 10 years ago, for the top-of-the-line databases, you’d have to spend, say, a million dollars a year to run it. You’d have to pay for the machines that run it. You’d usually have to have one or two people whose full-time job was to manage it. Today, you can get warehouses faster than that one that will cost you $20,000 a year, and you don’t have to have anybody fully managing it. I can go to AWS, push a button and pay tens of thousands of dollars for something that used to cost me probably a couple of million dollars a year. And it’s a better product.
You could make that same claim across the entire stack. Analytics tools have improved in that way. Data ingestion tools and pipeline tools have improved in that way. We’ve had these dramatic improvements in the quality of the technology, but the core problems that we’re trying to solve are often stubbornly persistent. One of those core problems we had 10 years ago was two people walking into a room and being like, “My dashboard says this,” and somebody else saying, “My dashboard says this.” They spend the entire meeting, rather than trying to figure out what to do, arguing about whose number is right. And we still do that, there still is a lot of that.
“Marketing and sales have been around for hundreds of years. We’re still in the early stages of figuring what it looks like for the data profession”
I heard a talk from the CEO of Databricks, one of these really modern warehouses driving a lot of this change. He was saying that one of the most common complaints you hear from customers is that people spend all their time bickering over numbers and which one is right. I think part of solving that problem will be continuing to build tools that can talk to each other and work better together, and systems that allow us to define things once so that if we look at a dashboard, we know it’s pulling from the same place. But part of that, also, is just going to be organizations figuring out how to work with data. Data’s still a relatively new thing. Most companies have started using it in a real way in the last 10, 15 years. We’re still figuring out how to make that work. There are still a lot of conversations in the community about the different roles we need, what they’re for, and who’s responsible for what.
Engineering wasn’t a practice that developed overnight. IT wasn’t a practice that developed overnight. Marketing and sales have been around for hundreds of years. We’re still in the early stages of figuring what it looks like for the data profession. I think it’ll be a combination of the tools we build and just everybody starting to realize, “This is the way this works, and this is the way this doesn’t,” before we can really get past what seems like foundational problems.
Liam: Absolutely. I think everyone from all kinds of industries will have their own stories. Podcast metrics, I think, are probably a good example of the complications with data. A download usually doesn’t equal the total number of listeners because an individual listener might have downloaded the same episode on multiple devices, or they might have downloaded it with the intention of listening to it but never got round to it. I know they’re trying to introduce a standardized way of measuring these things, which is probably going to happen across the board, I guess, for a lot of industries.
Benn: If you think about the basic web marketing metrics and how people run ads and stuff, we’ve started to standardize on some of that. If you go to your Facebook ad dashboard or your Google ad dashboard, those things are going to look pretty similar. They’re going to tell you more or less the same story because there’s been some standardization on how we actually talk about and figure out which ads are performing well versus not. You see this with some other things. You see this with SaaS companies, for instance. SaaS companies now have a standard book of metrics they all draw from. There are a few places where we’re starting to see this coalesce, but there will be a lot more of that over the coming years, where it’s like, how do we think about podcasts? How do we think about particular types of products? How do we think about measuring the performance of a support team? How do we think about all of these different things that are the data we now have so much richer information about but every company is still trying to figure out individually?
“We’ll be adapting from best practices instead of everybody trying to do this from scratch”
I think there will be some standardization around, “This is probably the best practice for thinking about these sorts of problems.” Companies will still obviously have some bespoke reporting that they need to do. This podcast may want to measure things differently than that other podcast because you may have different audiences or different goals. Everybody will have their own tweaks on it, but I think there will be a much better sense of, “Okay, this is the way we all agree works.” We’ll be adapting from best practices instead of everybody trying to do this from scratch. A lot of companies that start their data practices are often trying to build things from the ground up. I think we have a little ways to go before we get to the point where you have the scaffolding that we can all build on top of.
Web frameworks, in some ways, are an example of that. If you want to build a web product or software, there are pretty well-defined outlines you can draw from where you then add your own logic to make your application exactly what you wanted. Analytics just doesn’t quite have that. Everybody starts from zero rather than having that kind of core framework they can build on top of.
Liam: What would you say to startups daunted by data that are staring at it all and feeling a bit anxious about it?
Benn: This can happen at startups, but it can also happen at data-mature companies with individual analysts. It’s easy to stare at a blank page or at an overloaded Excel spreadsheet and think, “What in the world do I do?” That’s easy to do if you’re an experienced analyst just starting to understand a problem, it’s easy to do if you’re a company that doesn’t yet know what to do with data, and it’s easy to do if you’re a junior analyst who just joined somewhere and isn’t sure what to do.
“It’s okay to start with stuff that’s really simple. It’s remarkable how much basic information people will find valuable”
I think the solution for that is all the same – it’s okay to start with stuff that’s really simple. It’s remarkable how much basic information people will find valuable. I know a guy who, once a week, would have his data team meet so they would essentially share the things they learned over the week. Some of these things were stupidly simple. The company was a product that was built for businesses, so it was used Monday through Friday. They made a chart for how many people use it by day of the week, and hey, most people don’t use it on weekends and a lot of people use it on weekdays. You’re not exactly uncovering some incredible insight there. Everybody already knew that was how it worked, but seeing those numbers and exactly how much it dropped off on weekends – there were some weekends where it didn’t drop off very much, and some weekends where it dropped off a ton – generated a bunch of questions and curiosity around what’s driving that behavior. Why is that weekend one where people seem to use it a lot? What happened there? That was the seed for a lot more of, “All right, let’s continue to learn. Let’s continue to ask questions. Let’s continue to dig.”
That’s the way that I encourage people to start. Just start with the basic things, even if they’re assumptions you’re making. As soon as you start to look at numbers, you will see things that make you think and scratch your head and make you realize there’s so much more here that you don’t understand that you’re curious about. A lot of it is just allowing yourself to be taken by that curiosity and not saying, “I need to go uncover some amazing thing. I need to find that needle in the haystack that will change the trajectory of the company on day one.” You’re not going to find that right away. The way you’re going to find it is by just poking around, seeing things that are interesting, and letting yourself explore those interesting things when you find them.
Always tinkering
Liam: You’re clearly so passionate about data. I was just wondering where that came from.
Benn: Part of it is working in it for a long time and just getting to know it. Part of it, though, is that I think it’s fun to figure stuff out like this. I was talking to someone about flying from New York to San Francisco a couple days ago, and it’s a flight that I’ve made a good bit, and they had made it a good bit, too. They were saying that when they were flying back on a Delta flight, it felt way faster than when they were flying on a United flight. Why is Delta faster on this? And it made me think: are there some airlines that are just able to fly faster? It doesn’t seem like that would be true, but does that actually hold up? The Department of Transportation releases raw data on every flight in the US. Where it started, where it ended, what time it took off, when it landed – all of that stuff.
“I’m just naturally prone to want to play around with that and understand it. I don’t really have an explanation as to why that is other than I find it fun”
I just started playing around with it, trying to figure this out. I looked at different routes and was like, “Are there changes in the speed of different airlines? Are planes flying faster at different times of day, at different times of the year?” As you get into it, it just became interesting to see… I don’t know any of this stuff. I don’t know how fast planes fly or why they fly at different speeds on the same routes or whatever, but it was interesting. There were actually some airlines that were consistently a couple minutes faster. I don’t know why that would be. It was never by much, but it was basically like 90 seconds of difference fairly consistently between a couple airlines. I don’t know, maybe they just have different guidelines or something. That sort of thing, I think, is what makes me interested in it. I’m just naturally prone to want to play around with that and understand it. I don’t really have an explanation as to why that is other than I find it fun. That really drew me to the job in the first place. Then, once you get into it, you start to see all these other things that you could be doing that are fascinating and fun.
Liam: That’s great. What’s next? Have you any particular plans or projects for 2022?
Benn: I mean, continuing to do the Mode thing. I think there’s a lot of stuff on that front that we’re excited about, a lot of new things we’re building. The direction of the space is one we’re very excited about. Personally, last year I basically started writing a lot more on Substack, and that’s been fun. It’s been good to get some of the thoughts out about these ideas, have conversations with the people in the community and see where things go. We’re planning on being much more involved in that. I don’t know exactly where that goes, and part of this is, again, letting myself be curious about it, do the things I enjoy, and if I find stuff that’s fun and other people seem to enjoy, then giving myself the permission to continue to do it.
Liam: I was going to ask where our listeners can go to keep up with you and your work, definitely the Substack. I think it’s Benn.substack.com. Is that right?
Benn: That’s correct. The two places would be mainly there and Twitter. I’m not the most active Twitter person in the world, so Substack is better for some of the more interesting things. But my Twitter handle is @BennStancil, and there’s also LinkedIn if you want to connect professionally.
Liam: I’ve been reading lots of your posts and they’re great, so I would definitely recommend everyone go and subscribe to Benn’s Substack. Thank you so much for chatting with us, Benn.
Benn: For sure. Thanks again for having me. This was fun.