AI skepticism is giving way to curiosity among customer service teams
There’s a lot of talk in the customer service industry about support agents feeling “AI anxiety” – there’s a fear around the impact generative AI is going to have on jobs.
It’s understandable that change may make some people a little apprehensive, and that’s okay. There’s no doubt that the industry is going through massive disruption.
Leaders need to think about how they want to use AI to drive business success. AI will certainly help to cut costs, but we believe its value is felt just as much in the way it enhances customer and team experiences.
As we continue to pioneer AI-first Customer Service here on the Intercom Customer Support team, we’re realizing more and more that AI isn’t happening to customer service folks – it’s happening for them.
Empowering support teams
The reality is that AI tools are incredibly empowering for customer service teams. Support leaders who have already started adopting AI will probably identify with some of the following changes we’re experiencing:
- Support reps don’t have to deal with all of those boring FAQs that they used to have to answer over and over again – that feeling of tedium is lifted when there are fewer repetitious queries to handle.
- The support queues are freed up so your team will only handle really complex queries that truly need the human touch – support reps feel challenged and engaged more often.
- Support reps can spend time with each customer and really give them the care, attention, and help they need instead of trying to move through as many topics as possible. We’re finding a greater sense of achievement as we help each customer.
- The process of training and upleveling is made dramatically easier with AI tools – relevant information is now at your fingertips, helping you get up to speed way faster than previously possible. That sense of helplessness that new reps can feel for quite a long time is replaced with a sense of confidence.
- People who work in customer service can move into more valuable, strategic roles. The support role becomes a lot more challenging, interesting, and enjoyable. It’s deeply empowering to work at the cutting edge of technological adoption, and realize how many opportunities it opens up.
We’re detecting a widespread change in attitude around all this, especially since we announced Fin AI Copilot, a personal AI assistant for every agent. The skepticism is giving way to curiosity.
Your first 30 days
We’ve heard from many folks who are wondering how to get started with AI. Our advice? Just do it – you won’t regret it.
Here’s a quick guide to your first 30 days with AI.
🪜 Start small
At Intercom, when we first rolled out Fin AI Copilot, we tested it with a small internal group of Support teammates. This helped us figure out how best to integrate it into our existing troubleshooting and teammate workflows. This small group could report back to the larger team on what was working before we rolled it out to everyone else.
🧑🎓 Use it to onboard new hires (but set clear expectations)
Before, when new teammates were onboarding it took them a long time to search our help content and past conversations to find the right answer to a customer question. Now, Fin instantly provides the answer and instructions for them, which provides fantastic efficiency gains. But it’s important to set expectations with new hires that they shouldn’t just copy and paste answers. They still need to understand the job-to-be-done behind the customer’s problem and the solutions they’re sharing. The good news is that Fin can help them learn far quicker than before.
📄 Beef up and optimize your internal knowledge content
Fin AI Copilot pulls from your internal and external knowledge content like your dev docs, internal knowledge articles, as well as past conversations. At Intercom, we’ve talked lots about the importance of beefing up your external knowledge content. Now is the time to fill in the gaps and optimize your internal knowledge content to make it clear, accurate, and easily understandable for customers too.
📈 Measure efficiency gains (you will see them)
Fin AI Copilot will remove steps involved in troubleshooting issues and having to navigate a ton of different resources across multiple tools and docs. As a direct result, you’re going to see those efficiency gains, and it’s important to have some metrics and early signals to be able to assess and get a pulse check on that.
Fin AI Copilot is not like other new tools or workflows that require a ton of change management. You can get started quickly and easily, in a risk-free way. And you can see significant, early results with low upfront investment.
Any level of change can be daunting, but our own experience delivering AI-first Customer Service is making it clearer than ever – this change is more than worth it.
The ultimate guide to knowledge management in the age of AI
AI is revolutionizing the customer service industry. Our Customer Service Trends Report 2024 revealed that almost half of customer support teams are currently using AI, with more set to join them this year.
The benefits of AI-first Customer Service are incredible: 24/7 availability, multilingual support, major time savings, and fast, efficient resolutions for customers. But behind every great AI-driven support experience, there’s an unsung hero: knowledge management.
A comprehensive, well-structured knowledge management system is the power source of the AI machine. Whether you’re using a chatbot or AI agent to interact with customers, or an AI copilot as a personal assistant for your support team, these tools need an extensive pool of knowledge to draw from so your customers get accurate, up-to-date information – and your support reps don’t have to answer the same questions over and over.
In this guide, we walk through everything you need to know about effective, AI-optimized customer service knowledge management to help your team take advantage of all the benefits AI has to offer.
What is knowledge management and why is it so important?
Definition: Knowledge management is the process of creating, organizing, sharing, and maintaining knowledge in your business.
Your customer-facing knowledge base is a classic example, but your help center articles are just the tip of the knowledge management iceberg. In fact, knowledge management involves a range of activities such as:
- Creating resources like help center articles, blog posts, internal guides, or learning materials.
- Identifying gaps in your documented information.
- Implementing systems that make it easy for team members (both humans and AI) to access and use this information when solving customer problems.
- Developing workflows to ensure that your existing materials are continuously updated.
Why is knowledge management more important than ever in the age of AI?
Your knowledge base is no longer only for those intrepid customers who want to trek to your help center to self-serve – in the age of AI, it fuels everything.
That’s because your knowledge base is a crucial input for your AI-driven support experience – it’s the key to accurately answering complex customer queries, speeding up resolution and handling times, and delighting your customers.
Here are three reasons why knowledge management is on every forward-thinking support leader’s mind right now.
1. AI is only as strong as what you “feed” it
AI is only as good as the knowledge and content that it has access to. A lack of information, badly structured articles, or out-of-date documentation all prevent it from providing clear and correct answers to your customers, leading to poor customer experiences that degrade trust and fall short of their high expectations.
No large language model (LLM) knows your business like you do. ChatGPT doesn’t understand your customers’ needs, pain points, and use cases. That knowledge is unique to you and your organization, meaning you need to be the one to map it all out and feed it to AI.
2. Every investment of knowledge has compounding results
Making the switch to AI isn’t just adopting a new tool – it means adapting to a new ecosystem. And the sooner you start planting the seeds, the sooner you can start harvesting the rewards.
In less horticultural terms, every upfront investment you make in your knowledge base has long-term benefits. And whether you hire someone to do this work full time or give your agents time away from the queues each week, the ROI speaks for itself.
Think of it this way: say it takes 30 minutes to write a new help doc for a commonly asked question. That 30-minute investment results in:
- Hours saved for your support reps, who no longer have to spend time responding to that query because now AI can handle it instead.
- Calculate: Average time to compose a response X frequency of query = time saved for your team.
- Hundreds of satisfied customers who get an instant, accurate response to their question and don’t need to wait around for an available agent.
- Calculate: Number of customers who ask this query X average time to resolution = total time saved for customers.
- Data about your help docs and AI support experience for you to learn from, so you can make them even more effective.
- Monitor: AI involvement rate, resolution rate.
That’s a pretty good ROI for half an hour’s work.
3. Continuous learning leads to continuous improvement
When it comes to anything AI, the best way to learn is by doing.
A robust knowledge management process ensures you’re constantly getting valuable data to iterate on, so you can see what works and what doesn’t. This helps you to identify opportunities and areas for improvement that will have the biggest impact for your customers and your team, so you can train AI to be the best it can be.
But to start gathering this wealth of information and making these data-driven, customer-centric decisions, you need to start. As the old proverb says, “The best time to plant a tree is 20 years ago. The second best time is now.” The sooner you start getting insights about what your customers want and need from your customer service, the sooner you can deliver it.
What kind of information should you cover in your knowledge base?
The great thing about using an AI-powered platform is that it gives you data-driven insights to help you identify and prioritize which help content to create based on what customers are actually looking for. For example, with Intercom’s Fin, you get access to an “unresolved questions” report that gives you insight into queries that Fin was unable to resolve and enables you to pinpoint areas where new content is needed.
But if you’re just starting out, or are looking for a quick refresher checklist, here are some examples to get you started – as well as multiple ways you can leverage this content to get the most bang for your buck.
1. Troubleshooting and FAQs
- What it is: Troubleshooting and FAQ documents answer common queries or problems that your customers have, like “How do I update my billing information?” or “Why hasn’t my order shipped yet?”
- How to source information: Look for questions that come up again and again in your inbox, as well as topics your customers frequently search for (like “Billing help” or “Order updates”).
- Where to use this content: Knowledge base, AI agent/chatbot, AI copilot, proactive support like tooltips.
2. Quick-start product or feature guides
- What it is: Quick-start guides teach users how to efficiently set up products or features by following a series of sequential steps, such as, “Create your workspace,” “Invite your team,” or “Run your first report.”
- How to source information: Talk to your customer success team or onboarding specialists to learn their recommendations for getting started, or ask your product team to document this process when they’re building new features.
- Where to use this content: Knowledge base, AI agent/chatbot, AI copilot, product tours.
3. Troubleshooting and advanced guides
- What it is: Troubleshooting and advanced guides help users get even more from your product or feature once they’ve mastered the basics, leading to next-level adoption.
- How to source information: Get input from internal experts like product managers, R&D, and customer success managers.
- Where to use this content: Knowledge base, AI agent/chatbot, AI copilot, targeted messaging aimed at advanced users (sent in-app or via email).
4. Best practice tips for specific use cases
- What it is: These are tips aimed at specific cohorts of users who have similar jobs-to-be-done, like engineering teams or startups.
- How to source information: Use a mix of hypothetical examples based on your target audience (your marketing team can help here) and conversations with real customers about their goals and workflows.
- Where to use this content: Knowledge base, AI agent/chatbot, AI copilot, tailored webinars, learning courses.
Create a knowledge management process that fuels your AI with these 5 steps
1. Find the gaps in your existing knowledge base
Do an audit of your existing content
The first thing to do is review what materials you currently have. This is for two reasons: firstly, you need to make sure that the AI isn’t learning from out-of-date information, and secondly, it identifies where the current gaps are.
At Intercom, we had over 700 live articles to audit before feeding them to our AI agent, Fin. To do this, we divided the articles into product areas and gave relevant teams a week to check, update, or retire each article. Sharing the ownership like this made it a real team effort, and broke a mammoth job into achievable tasks.
Put yourself in your customer’s shoes
Walk yourself through the same steps that your customer will take when they look for help, including their first encounter with your AI-driven support experience.
“As part of that first deployment, test it yourself, and make sure that you actually experience the experience that your customer is going to have,” advises Declan Ivory, our VP of Customer Support. This will help you test out the quality of the answers and spot any missing topics or keywords in your content.
Seek input from your teams
When auditing and identifying gaps in our content, we didn’t just rely on our customer support team: we took an “all hands on deck” approach. By including your product and engineering teams in this process, you can get expert advice from the people who know more about your product than anyone else (because they built it!). Your sales, marketing, and customer success teams will also have unique insights about what matters to your customers and what they’re trying to achieve.
Use the initial data from your AI agent or chatbot
After 30 days of using an AI agent or chatbot, you’ll have enough data to see where it’s able to successfully resolve questions versus where it’s getting stuck – and why. Dig into that data to find areas to beef up (i.e. topics that don’t have enough content for AI to handle and get handed over to support reps) or articles that need improvement (i.e. conversations that have poor resolution rates or low customer satisfaction scores).
⚡ Pro tip: If you’re just getting started, consider testing your AI agent/chatbot with a segment of your customers first to get this initial data. Then, once you’ve addressed any immediate gaps, move on to a wider rollout.
2. Make a plan to address the backlog
Prioritize which content to update or create first
By now you’re probably bursting at the seams with amazing help content ideas from every corner of the company. Next step: deciding where to start.
When you’re prioritizing content, what you’re really trying to do is find out what’s driving volume for your customer support team and identify the easiest wins that will reduce that volume. To help you manage your resources and work on things with the greatest impact, try these tips:
- Look at data and metrics from your conversations to see the most asked questions, which queries have the longest handle time, and which conversation topics have lower customer satisfaction (CSAT) scores, then create or improve content around those topics.
- Prioritize topics based on the value they’ll bring to the business. For example, create documentation for features on higher tier plans before free ones.
- Use reporting to find searches with no results, or filter by “Last updated” to find help articles that haven’t been updated in a while and may need a refresh.
Decide what information you’ll feed the AI
When sourcing knowledge for AI to use, cast a wide net – because you probably have more relevant content than you realize. We quickly learned that almost any and all information is useful once it’s framed the right way, so leverage what you already have.
For example, with Fin, you can use:
- Your help center
- Past cases and conversations
- Inbox suggestions
- Saved macros
- Snippets
- Internal documents (like Notion or Guru knowledge bases)
- PDF files
- Website pages
- Your blog
Allocate time and resources
Be intentional about carving out time to work on your help content. “The way we do it at Intercom is that we have something called a ‘Special-T’,” says Anthony. “This is a dedicated team of frontline reps, a mix of our support specialists and engineers who are actually spending time out of the inbox every single week. We typically allocate maybe 5–10 hours per individual contributor,” he shares.
Together, this group works with the knowledge manager (that’s me!) to build out our backlog of content.
⚡ Pro tip: These projects are a great way to help your support reps upskill and develop into the exciting new roles AI is creating in customer service.
3. Monitor KPIs to measure success
Review key performance indicators
Once you’ve started using AI, track KPIs and business metrics to measure the impact it’s having. Some relevant KPIs to watch out for include:
- Customer satisfaction (CSAT) score, for both bots and humans
- Resolution rate
- Self-serve rate
- Chatbot involvement rate
- First response time
- Number of handovers to human support reps
All of these metrics help you spot which content is performing best – and where you can improve your knowledge management process.
Put your learnings into action
Ideally, you’ll see amazing results straight away (woohoo!), but it’s highly unlikely that you’ll get everything right immediately. There’ll be some problems AI can’t solve yet, some workflows that need adapting, and some users that didn’t get the answer they wanted but never followed up to let you know (resulting in a “false positive” resolution).
All of this is good, because it gives you real data about what your customers need and value to achieve “true” resolutions. The most useful insights and impact will come from these resolutions. Do the work, iterate, and keep monitoring and reporting.
4. Audit, audit, audit
Build ongoing maintenance into your workflow
Knowledge management is a process. It doesn’t end once you’ve published a certain number of help articles.
As your product, customers, and business goals evolve, so too should your help content. This means you need to build maintaining, updating, and creating new content into your team’s workflow on an ongoing basis – not just in the mad rush before a new feature gets launched.
Map out a plan for updating your content that outlines:
- Who is responsible for refreshing or creating new content.
- How often existing content should be reviewed so it doesn’t become stale.
- When they should do this (i.e. for one hour per day, every Friday, monthly, or whatever cadence makes sense for your team).
Develop a system to log requests for new content
Encourage the cultural shift to a “knowledge management” mindset by making it easy for everyone to share ideas for new or improved help content. Create a simple system for team members to log content requests, so you can capture insights from all customer-facing and product teams – and address customer needs from every angle.
5. Build knowledge management into future launch plans
Make knowledge management an essential part of product development
Depending on your industry, you might be building new features or ship new products, and it’s crucial that you create high-quality help content for them should be an integral part of your launch checklist. Work with your product team, product managers, and product marketing managers to build out your launch content, then review customer conversations after going live to spot opportunities for additional resources.
Best practices for AI-friendly knowledge management in 2024
Use the terms your customers are using
Getting the language right in your help documents is important – and tricky. Language is diverse, and varies by location (“cilantro” versus “coriander”), dialect (“hoagie” versus “sub”), and even different types of users (a marketer might call someone a “lead” while a salesperson might call them a “prospect”). Analyze your search data to discover what words your customers use, and speak their language.
⚡ Pro tip: Introduce AI to different groups of users, like power users and customers on a free trial. This reveals variations in wording when asking the same questions, which you can incorporate as you create materials for different audiences.
Simplify your language and remove ambiguity
Luckily, machine-friendly language also means people-friendly language. Remember that you’re not just writing for AI, but for real people with all different technical abilities and backgrounds. Keep your language as simple as possible: avoid jargon, spell out any acronyms, and explain key terms.
📺 Learn more about avoiding ambiguity in your support content →
Create a consistent, trustworthy, and on-brand experience
Brand consistency is crucial for building customer trust. It ensures that people feel like they’re talking to one company, no matter which customer service channel they use. To achieve this, make sure that product and feature terminology is consistent across every touchpoint, proofread for spelling and grammar, and use the same formats when creating new help docs to keep them cohesive (templates are super helpful here).
Add context to your answers
“If you’ve got an FAQ document today that a human can interpret and you’ve got simple yes or no answers in there, the machine won’t interpret those answers in the same way that a human does,” explains Declan. “You’ve got to expand on what you mean when you say ‘yes,’ what you mean when you say ‘no.’” To do this, we recommend restating the question in your answer; this gives AI extra context and clarity that helps it learn.
📺 Learn more about optimizing your help center answers for AI →
Add text to images and videos
Showing as well as telling is great – but AI can’t parse videos or images, so always include explanatory text alongside them. Not only is this more accessible for AI, but it’s more accessible for your audience, too, ensuring that users with visual or auditory impairments aren’t left out.
Create a scannable structure with formatting
Use headers, bulleted lists, and tables to organize your information and make it easier for AI (and human readers) to quickly find the information they need. H1s, H2s, and H3s are all helpful when used correctly – but don’t have large chunks of information below H4s, as it’s difficult for AI to find information there.
📺 Learn more about using rich formatting in your help content →
Include contact details for customers who need them
Including contact information reassures customers that if AI can’t answer their problem, they’ll still get the support they need. Just make sure to include context for the information you provide so that it’s crystal clear when and how to use them.
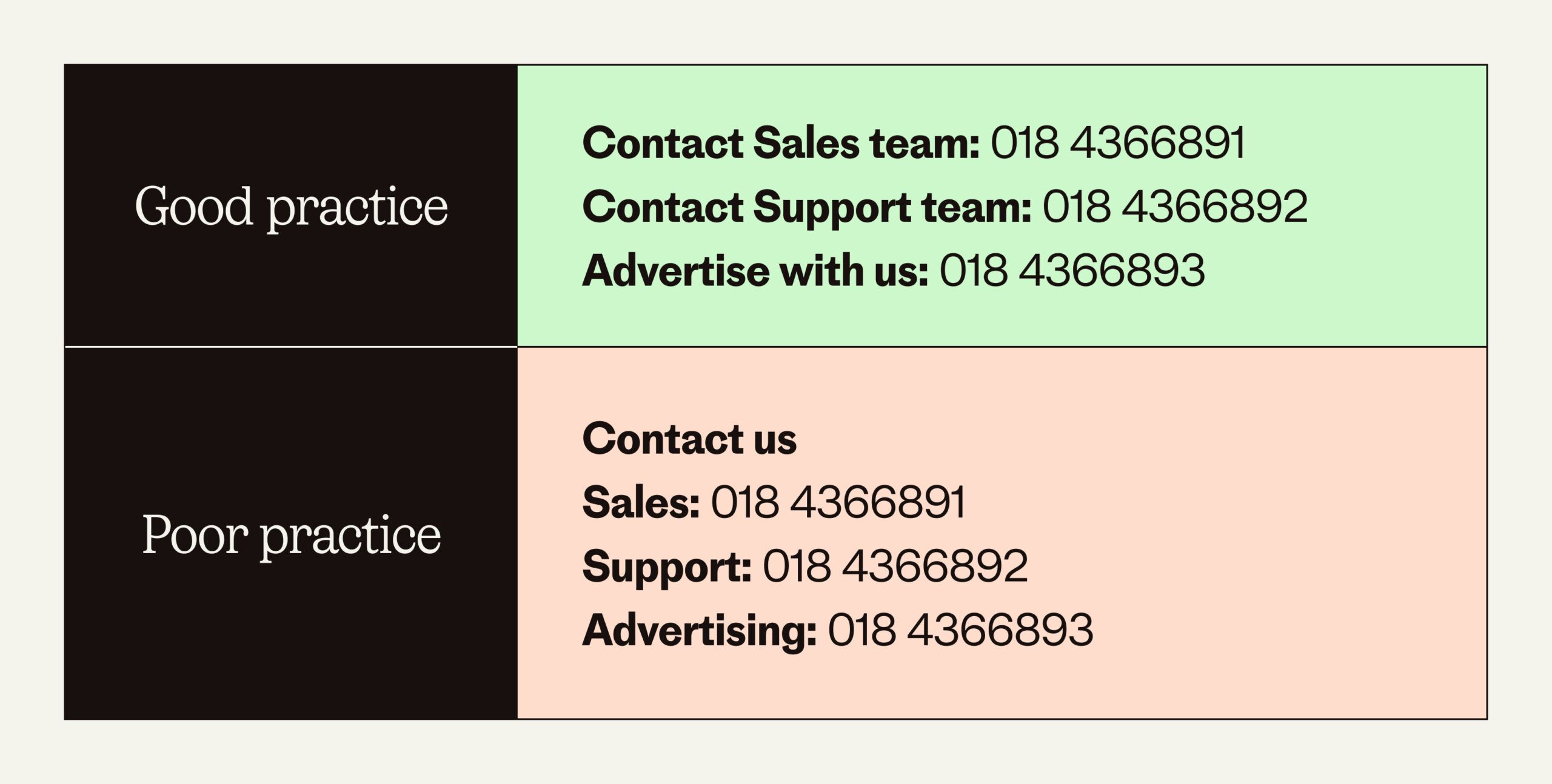
Include context for any contact details so your users know the best channel for their needs
Collect bitesize information in FAQ articles
If you have small pieces of information that don’t need a full article, compile them into a list of FAQs. These can be some of the highest volume, most repetitive questions, so this way, AI will still be able to find the answer.
Clearly identify who the content is aimed at
If you have different help content for different types of users – for example, customers on different price plans who may not have access to all the features mentioned – make sure each piece of help content clearly references who it’s for. You can also use behind-the-scenes audience targeting rules and workflows to help surface the right content for the right users and deliver a more personalized experience.
Use an AI-first customer service solution to get (and action) more data
When you’re using a consolidated AI-first customer service platform, everything becomes part of a powerful cycle. All your customer support data is in one place, making it easier to analyze, monitor, and iterate on the support experience and your knowledge base as a whole – simply, quickly, and securely.
Power up your AI with a future-ready knowledge management process
AI is vital to creating an industry-leading customer experience – and to perform efficiently, it needs to be underpinned by a comprehensive, AI-friendly knowledge management process.
Ready to put these tips into practice? Start a free trial of Intercom’s AI-first customer service platform and start turning your knowledge base into resolutions.
There’s no going back – the new era of AI-first Customer Service has arrived
The era of AI-first Customer Service is here, and it will lead to happier customers and more strategic customer service teams.
It’s already clear that AI will reshape society, just as major technology breakthroughs have done in the past. Technology doesn’t go backwards. The car created shopping malls, suburbia, and McDonald’s. The internet created instant communication, real-time shared events, on-demand everything, for anyone, anywhere on earth. We’re not going back to riding horses, nor printing out photos to post to our family across the world. AI is as big an invention as the internet, and possibly as big a change as the industrial revolution. We’re only 18 months into a 10+ year cycle.
“Almost all customer questions, in all customer channels, will be excellently answered by an AI Agent”
Customer service is one of the earliest areas to be fundamentally changed, because AI happens to be excellent at the things customer service people do today. This transformation is already happening at a faster pace than anyone predicted. Based on results we’re seeing from our customers (some already have up to 80% of customer questions being accurately answered by AI), we can now confidently predict that in the very near future, almost all customer questions, in all customer channels, will be excellently answered by an AI Agent.
This is real. This is happening now. When an AI Agent can answer the vast majority of customer questions, this fundamentally changes the nature and makeup of a human support team. The work changes, the economics change, the metrics change. Nothing is left untouched. With AI-first Customer Service, support teams work on higher value, more complex customer questions, and they invest most of their time analyzing, managing, and improving the AI system, so it can deliver even better results for customers.
What is AI-first Customer Service?
At the highest level, AI-first Customer Service involves first seeing if AI can resolve the problem directly for the customer, and if not then seeing if AI can help the agent solve the problem.
AI-first Customer Service means customers will get:
- Instant, excellent answers to almost all their questions, delivered by AI.
- And when it isn’t instant, they get deep, attentive, personal, human service because agents have more time and have AI to help them.
AI-first Customer Service has three components that work together in a single system:
- AI Agent first: Customers experience AI first. Instant, accurate answers.
- AI Copilot first: Human agents experience AI first. Instant always-on help that comes to them.
- AI Analyst first: Leaders experience AI first. An AI Analyst proactively delivering insights to them.
AI-first Customer Service is both a product change and a mindset change.
Businesses that change their mindset and adopt AI-first Customer Service will deliver remarkable customer experiences. Those that don’t will get left behind. At Intercom, we’re pioneering this new way. Let’s dig into the details.
AI Agent first: Customers experience AI first – instant, accurate answers
When customers have questions and problems, they want answers and resolutions, as quickly as possible. Talking to the customer service team is a means to an end. The best customer experience is an instant, accurate answer, or immediate action taken to resolve the problem.
AI can already answer many questions and resolve many problems, and oftentimes better than a human can because AI is always available. AI answers instantly, works 24/7, and speaks every language.
As the technology improves, and forward-thinking support leaders learn how to best set up AI-first Customer Service, AI is simply going to get better and better at answering customer questions.
This transformation is already happening. We launched our Fin AI Agent in May 2023. On day one, the average resolution rate was 28% of customer questions. Less than a year later, the average resolution rate is 46%. Thanks to AI and feature improvements we’ve built, and our customers learning how to optimize their content and data, our Fin AI Agent went from resolving a quarter of queries to a half of queries in just one year:
Aside from the pace of improvement, what is striking is that customer satisfaction scores remain high. In fact, for some customers who have deployed Fin AI Agent, their human support satisfaction has increased, because their human team has more time to spend with customers on harder problems.
“For some, AI is already answering up to 80% of their customer questions, without a drop in customer satisfaction”
46% is the average resolution rate across our customer base. For some, AI is already answering up to 80% of their customer questions, without a drop in customer satisfaction. For the majority, it’s answering 30-50% with almost no upfront investment.
AI Copilot first: Human agents experience AI first – instant, always-on help that comes to them
When an AI Agent can answer the majority of customer questions, only the hardest ones get passed to the human support team. These questions require research, troubleshooting, and can take time.
AI is excellent at helping with these tasks. AI can ingest far more content and information than any human can, including all historical customer support conversations, and can offer suggestions and advice to help the human agent. This is the AI Copilot, a personal assistant for every single human team member. Copilot is ever-present, connected to all data and systems, and provides information and troubleshooting advice in real time.
Copilot removes all the heavy lifting of using slow, outdated systems, digging through many docs and old conversations, and makes team members faster and more efficient. Copilot also means instant ramp time for new agents, and other agents needing to know new things. Because the AI Copilot has access to all conversation history and all satisfaction scores of those conversations, it can provide agents with not just the correct answer, but the best answers to questions.
We launched our Fin AI Copilot into beta in April 2024, and early customers are already seeing up to 31% efficiency improvements.
Copilot also teaches itself to get better. It learns from what human agents do and don’t say, as all new conversations and resolutions get fed into the AI Engine. As Copilot learns what the right answers are to harder questions, it tells the AI Agent, so the AI Agent can answer next time. It’s an intelligent system that gets better the more it is used.
AI Analyst first: Leaders experience AI first, with insights proactively delivered to them
The transformation of customer service is not limited to customers and human agents. AI is also excellent at many of the things support leaders need to do, like querying data to understand insights and performance in real time or retrospectively compiling reports and charts, and training new human agents with real-time feedback and suggestions on best practices. And AI can do all this far faster than any human support manager or leader.
Just as human agents have a Copilot, support managers and leaders will have an AI Analyst to help them, to answer their questions, but most importantly to proactively bring them new insights which they can act on to improve service quality.
“AI will elevate the role of a support manager to be much more strategic”
For the first time ever, support leaders have access to knowing the quality of 100% of their customer conversations, because rather than rely on customers answering customer satisfaction surveys, AI can understand whether a question was successfully resolved, and understand the sentiment of that customer. The AI Analyst proactively provides a holistic view across all conversations and suggests clear areas of improvement.
AI will elevate the role of a support manager to be much more strategic. Rather than wrangle the day to day of support operations, they will design and optimize the overall AI-first customer experience.
At Intercom, we already have early AI Insights features live, with much more to come and an AI Analyst product in the market later in 2024.
Data and knowledge content will become strategic priorities
The AI-first Customer Service platform also has a data and knowledge layer that AI uses, learns from, and improves. This centralized hub includes internal and external data, and internal and external content sources.
The quality of the data and knowledge layer is foundational to the quality of support. Intercom customers who have invested in better data and better content have seen huge improvements in the resolution rate of their Fin AI Agent. In fact, on many occasions we have seen customers think that our Fin AI Agent is giving wrong answers only for it to become clear on investigation that the underlying content is out of date or wrong.
This is the power of an AI-first Customer Service solution on one single, seamless platform. It can easily surface suggested areas of improvement, and the system gets better and better. Because it’s built together, Intercom’s AI-first Customer Service platform improves much faster than one where different tools from different companies are stitched together.
AI-first Customer Service is better, faster, cheaper
AI is already transforming customer service. This is not speculation, the evidence exists right across our customer base.
Customer service will be one of the earliest areas to be completely transformed by AI, and the transformation will be incredibly positive.
The customer experience will be better. Customers will get instant, accurate answers almost always. Customer satisfaction will soar. First response times will be decimated.
When customers don’t get instant answers, they will get personal, attentive human service, where the human support team work on much more interesting problems, and have more time to give excellent support.
Customer service leaders will have much more strategic roles to play. They will work on designing a system that not only delivers excellent service, but that delivers new insights to the business about their customers.
And leaders will be able to reshape their team, moving people into new roles like improving content, AI system design, and doing much more with less. Support teams will be small and mighty.
This is an adapt or die moment for customer service
Customer service teams will adapt AI-first Customer Service soon or be left far behind. We already see early adopters reaping huge rewards with industry-leading customer service and soaring career progression. Some may think they are crazy at first, but they will win. Sitting on the fence is not an option. The pace of AI technology advancement is too extreme – it will quickly leave businesses that resist behind.
Intercom is pioneering AI-first Customer Service. Join us and we can build a better future together.
Introducing Fin AI Copilot: Increase agent efficiency by 31%
Intercom is leading the industry into a new era of AI-first Customer Service, and today we’re excited to reveal our latest breakthrough innovation – Fin AI Copilot.
When we launched our groundbreaking Fin AI Agent last year, it set the standard for generative AI customer service experiences – it has already helped customers with more than 8 million queries and resolves up to 80% of conversations instantly.
And now, for the more complex questions that require an agent, we’re applying the same innovative AI to make your teams even more efficient. Fin AI Copilot gives every customer service agent their own AI assistant in the inbox, helping your team find instant, accurate answers based on your support content. By pulling from multiple content sources and making it easy to verify every answer, it empowers agents to deliver consistently remarkable customer experiences.
Product demo of Fin AI Copilot
A personal AI assistant for every agent
Historically, customer service teams have had to sift through multiple sources and various internal tools – often opening numerous windows and tabs – to find relevant information to resolve customer queries. With Fin AI Copilot, those fragmented searches and inefficiencies will disappear. One of our early testers, Lightspeed, has already seen a 31% increase in agent efficiency with Fin AI Copilot.
Here are just some of the ways Fin AI Copilot will change the game for support agents and leaders:
The best answers from conversation history
This cutting-edge new feature is the only AI assistant that can generate answers using your agents’ past conversations. Up until now, this goldmine of data has been untapped, but Fin can use it to customize and enrich answers with insights drawn directly from your team’s history.
Of course, not every conversation from the past is going to be relevant, so you have the power to determine which conversations contribute to the learning pool, ensuring that Fin only draws on the knowledge of your most experienced agents.
Trusted answers from any content source
Fin AI Copilot connects to an array of both internal and external content. From your help center, conversation history, and internal articles to public URLs, PDFs, and even integration with platforms like Notion, Guru, or Confluence, Fin sifts through all of your support content to deliver the best possible answer, every time.
With all those sources, it’s more important than ever for an AI system to be transparent about what content it’s referencing when suggesting an answer. That’s why it provides links to the sources which can be previewed directly inside the inbox, so agents can easily cross-reference and validate the answers.
Expert training, troubleshooting, and guidance
Fin AI Copilot is like having an on-demand expert for your agents, ready to guide them through next steps, troubleshoot complications, and tackle follow-up inquiries that would typically require input from teammates or supervisors.
By drawing on your internal articles, it can provide agents with instructions on how to handle queries. This is especially useful to onboard and train new agents – they can simply use Fin to get up to speed with handling unfamiliar situations. And best of all, that guidance is immediate, and in-context.
Deep insights, complete oversight
Adapting to this fundamentally new way of doing customer service will require careful monitoring to ensure standards are staying high. With Fin AI Copilot, you can keep a close watch on how your support teammates are using Fin to continuously enhance the quality of your customer service.
Part of that improvement process will involve managing the source content. We have ensured you can easily manage and restrict Fin’s access to specific content, tailoring its knowledge base to suit your evolving needs.
The impact of Fin AI Copilot on how teams are able to provide support is dramatic – for instance, Deliverect has already seen huge response speed increases among agents using the feature.
Welcome to the world of AI-first Customer Service
Fin AI Copilot will fundamentally change how you work inside the inbox. Soon, you’ll never have to answer the same customer question twice.
This is just the latest innovation we’re bringing to market – but there’s far more to come. Intercom has the most advanced and effective AI product offering in customer service. And it will just continue to get better.
Intercom’s AI is built into the foundation of our complete customer service platform – it dramatically improves the CS experience for customers, support teams, and support leaders.
We are inventing the era of AI-first Customer Service – it’s happening right now, and it’s happening faster than you can imagine.
Response Time: Vol. 25
You satisfy your customers, but can you satisfy our curiosity?
With Lucía Achigar, Support Team Lead at Bunker DB.
Please tell us a little bit about your company and what you do there.
Bunker DB is a martech company, born in Uruguay almost 10 years ago and now present in most Latin American countries. We promote intelligent, simple, and fully automated marketing solutions. I’ve been working here for eight years, nowadays as Support Team Lead.
What word or phrase in customer service jargon should be retired?
I think we should definitely retire old-fashioned clichés, especially the ones that appear to value the customer and their inconvenience, but are just a means to gain time or are simply put there by protocols. We should start having real conversations with customers, not following processes, protocols, or handbooks.
What’s the most valuable thing that working in customer service has taught you?
Throughout the years, I’ve learned that people really value when you use your brain. I mean, not everything can be solved by following a couple of steps; sometimes you need to make use of the different tools you have at hand to achieve what the customer needs.
Describe the essence of great customer service using only three words.
Empathy, resourcefulness, collaboration.
Which movie robot would you choose as your AI sidekick and why?
Baymax! Although its purpose is to take care of general health, I think its input would be amazing to manage angry customers and find creative solutions.
What can you do that a bot will never be able to replicate?
We can think of creative solutions, making use of available features to solve problems we hadn’t thought of before.
What’s the most embarrassing thing you’ve ever said/done to a customer?
In a conversation a couple of years ago, we weren’t able to offer an answer right away, so I began my message with “I’m afraid,” and it got sent before I could finish the sentence!
“We should think about whatever problem the customer brings to us as if it were our own”
Do you identify more with the title “customer support,” “customer service,” “customer success,” or “customer experience,” and why?
Our team identifies with “customer success.” Support is a part of that, and it aligns with the overall company vision. Bunker DB is a tool to make your work in marketing better, more intelligent, simpler. In short, we want to see our customers succeed.
What’s the one piece of advice you would give to your peers in the customer service industry?
To be empathetic, meaning just a little more than understanding our customer’s issue. We should think about whatever problem the customer brings to us as if it were our own – with the tools I have, how would I go about solving it?
What’s your greatest productivity hack?
Double monitors and good music.
If customer service was an Olympic sport, what would be the main event?
Sprint racing to keep SLAs in check.
What’s the best thing a customer has ever said to you?
Not that long ago, a customer expressed that they wished they worked with us.
What do you wish people knew about working in customer service?
We really, really care, and we feel awful when we can’t help.
If you wrote a book about your experiences in customer service, what would the title be?
“The customer service paradox: Advocating for a product we only get complaints about.”
What’s the strangest thing a customer has asked you?
Not once, but twice, people got in touch with us to try recovering their personal social media accounts.
What’s your most-used emoji in customer chats?
🙌🏽
If you’re interested in being featured in our Response Time series, you can share your insights on customer service – and movie robot sidekicks – with us here.
Customer service trends on tour: Bringing our 2024 report to life
Every year, we survey thousands of customer service professionals to get a pulse on what’s happening in the support space and publish our findings in our annual Customer Service Trends Report. And while creating the report is one half of the magic, bringing it to life with the help of our team, customers, and peers is the part I love the most.
For the 2024 edition of our report, we brought the customer service trends on tour. From global webinars to in-person events in Paris, New York, and London, we’ve had a blast unpacking the data and insights and getting our speakers’ and audiences’ takes on what’s happening in the customer service space.
Here’s the rundown of the Customer Service Trends 2024 tour and the stops we made along the way – as well as details of where you can catch up on some of the action in case you missed it.
Discussing the future of customer service
Across all of our webinars and events, we had a ton of insightful, engaging questions and conversations about how customer service is being transformed, and how these changes are being felt in our companies and wider industries.
The CS landscape is evolving so quickly, and we’re all learning as we go. It’s more important than ever for us to be having these conversations, sharing strategies and tactics to set our teams up for success in this new era of AI-powered support, openly discussing what’s failed and isn’t working, and paving the way forward together.
Here are some key takeaways from the discussions:
- The demand for instant, efficient support is on the rise: AI’s role in customer service is expanding quickly, and now that customers are getting used to super fast responses and resolutions, it’s what they expect. This isn’t going away and AI will be key to meeting customers’ expectations and staying competitive.
- Start small and iterate: Taking a phased approach to rolling out AI can help you make a smooth transition to new ways of working. For example, you can initially give AI lower-complexity issues to deal with, monitor its responses to spot any gaps in your knowledge base and optimize your content, and gradually increase its involvement rate in more complex conversations as you go.
- AI is there to enhance, not replace human interactions: “Is AI going to take my job?” has been a top-of-mind question for a lot of support folks since ChatGPT arrived on the scene. The answer is mostly “no.” Support roles are evolving, new roles are being created, and the main parts of support jobs that AI is coming for are the monotonous, repetitive parts. This will free up support teams to focus on more complex tasks and create value in other impactful areas for their businesses.
- Tech stacks and metrics need a reboot in an AI-first world: Last-generation tools and old success measures aren’t going to cut it in this new age of customer service. An AI-first approach will require a modern, complete customer service platform with AI at its core – not added on to the edges.
Worldwide webinars
This year we hosted two webinars, spanning time zones across Europe, North America, and Asia Pacific. These webinars were jam-packed with insightful conversations with customers and viewers about the trends that are resonating most with them, and how they’re navigating the once-in-a-generation shift to a world of AI-powered customer service.
I was thrilled to join the panel of our first 2024 Customer Service Trends webinar and dive into this year’s report with Matt Dale, Founder of Moxie CX, Allie Talavera, CX Program Manager at AppFolio, and Jared Brier, Founder and Co-CEO at AKKO.
You can catch up on the recording of my fun conversation with Matt, Allie, and Jared here
For the second installment of our webinar series, Intercom’s Sales Development Manager Dominic Bower hosted a conversation between our own Director of Customer Support Operations Anthony Lopez and Scott Donnelly, Customer Operations Enablement Manager at SiteMinder.
Full-house events
We hosted three in-person events in New York, London, and Paris to explore the trends and insights we shared in our Customer Service Trends Report for 2024.
Just check out these lineups.
🇬🇧 First stop: London
Two of our Intercom teammates – Eric Fitzgerald, Manager of Customer Support, and Peter Dalton, Senior Relationship Manager – took to the stage in London with Deborah Corless, Director of Customer Experience at Numan, and Nick Hills, Head of Support at Birdie.
🇺🇲 Second stop: New York
At our next stop in NY, I was joined by Chris Van Heran, Senior Manager of Business Systems Strategy at Octane, and JT Vega, Director of Product Support at Labelbox.
🇫🇷 Last stop: Paris
At our final stop of the tour, Intercom’s Senior Manager of Customer Support Franka Martinovic and Customer Success Manager Laurene Gachon sat down with Hilary Lawrence, Customer Support Operations Manager at Agorapulse, Auguste Guerlay, Head of Partnerships at Onepilot, and Clarisse Mehrain, Customer Care Team Lead at Choco.
Continuing the conversation
It’s been an awesome time since we published our Customer Service Trends Report back in January – in the best way possible. It’s been a ton of fun getting to unpack all of the trends, data, and insights from this year’s report with so many incredible folks as we figure out how to tackle the immense opportunities ahead together.
To everyone who joined us, whether virtually or in person, thank you. Your engagement, questions, and insights have been true highlights of the 2024 customer service trends tour.
Here’s to continuing the conversation. Until next time 👋
(PS: Cannoli work really well as swag, just in case you were wondering.)
Farewell to the customer service Frankenstack
You’ve probably met the Frankenstack – a friendly monster made with the best of intentions and from a concoction of different support software and tools.
I talked about Frankenstacks recently on The Ticket podcast with our VP of Customer Support, Declan Ivory, and our Director of Human Support, Bobby Stapleton. I wanted to clarify one thing: my issue with Dr. Frankenstein’s monster is not that he’s assembled from different components. It’s that he’s ugly (or put more politely, his individual parts don’t work seamlessly together).
In real terms, it’s not that I believe a single tool will do everything for your customer service team. It’s that the experience using these tech stacks is clunky, inefficient, and suboptimal, and we’ve tolerated it for a bit too long. With recent advances in AI-powered customer service, support teams don’t need to put up with disconnected tools anymore.
How did the Frankenstack emerge?
Much like Frankenstein, these ugly tech stacks don’t appear overnight – they sneak up on you. If you’ve worked in a customer service team, you probably know what it’s like to be viewed as a “cost center” with an unrelenting focus on response times and reducing spend. This doesn’t leave a lot of time or money to invest in corralling our tech.
On the one hand, hundreds of great tools emerged in the last decade to solve niche, but important, problems – and I’m grateful for them. That said, getting all of them to work harmoniously together is a huge lift. Pair this with limited bandwidth and:
- New tools are bolted on to existing support systems and end up requiring extra steps, logins, and another UI to learn. This inflates how long it takes to troubleshoot and makes your support team less efficient.
- Duplicative toolsets emerge and end up being underutilized, leaving your team with 2-3x the cost with little ROI (return on investment) to show for it.
- Your backlog of user feedback gets bigger and your team becomes more and more dissatisfied, impacting your team and customer experience.
What’s the solution? Rally your tech behind one platform and let AI fill in the gaps
1. Switch to an AI-first platform
First, migrate to a platform that makes it easy for you to streamline your tech stack – one that integrates with your tech easily but has its own core AI features. Here are just two of the ways we do this on our own team at Intercom:
- Our AI chatbot, Fin, works with our Messenger, learns from our Help Center, and has its own reporting for us to monitor performance – all in Intercom. This makes testing and improving our bot quality 10x easier.
- We use Intercom’s integration with Unbabel to help our team help customers in other languages. Fin can also provide multilingual support to customers in 45 languages.
2. Use AI to increase efficiency and improve the customer experience
Next, find and use AI features to save time and money without compromising the customer experience. Here are two recent examples of how we did this – and continue to do – this at Intercom:
- We used AI to analyze 500,000 conversations and help us understand key trends across our customers’ issues and questions. Imagine reading these manually.
- When handing things off in an escalation, our team uses Intercom’s Fin AI Summarize feature so that the next rep easily understands what’s going on – this is especially useful for long conversations.
Imagine a world where your tech stack saves your team money, features and tools work seamlessly together, and your team has more time to create white-glove customer experiences. We’re building that future at Intercom. 😉
Response Time: Vol. 24
You satisfy your customers, but can you satisfy our curiosity?
With David Henderson, CEO of Tubular.
Please tell us a little bit about your company and what you do there.
Tubular is an intuitive CRM focused on relationships with leads and key business contacts. Tubular was built to streamline your sales by helping you better organize your leads, close your deals quicker, and create sales reports in seconds. I acquired Tubular in January 2023, and I’m excited about where it’s going in the CRM marketplace.
Which celebrity would be really great at your job, and why?
Matthew McConaughey. Matty has it all – smooth, wise, Mr. Chill, not to mention looks. He’s just like me, lol.
What’s the most valuable thing that working in customer service has taught you?
No one cares how much you know* until they know how much you care!
*About your industry, your product, how connected you are, etc.
What’s your greatest productivity hack?
Activity beats everything. Turn off the noise and just do it.
What gif best describes your mental state right now?
What’s the best customer service you’ve ever experienced?
Apple. But the early 2000s Apple when they actually cared and did whatever they could to make you happy.
What’s the worst customer service you’ve ever experienced?
My chippy one night in Belfast – no craic/banter, no eye contact at all. Nothing.
Which movie robot would you choose as your AI sidekick and why?
Number “Johnny” Five from Short Circuit – what a dude. Childhood fave.
What’s the strangest thing a customer has asked you?
Did you get a First Class Honors at university … mmm, what? (Yes, I did, by the way.)
What can you do that a bot will never be able to replicate?
Play the guitar, ukulele, and tin whistle as poorly as I can.
What do you doodle when you’re on video calls?
Cubes. Many, many cubes. Sometimes pyramids if I’m killin’ it.
What’s the one piece of advice you would give to your peers in the customer service industry?
Keep going. Learn as you go. Have fun. Make new friends. Oops, that’s more than one.
What book are you reading at the moment?
Too many. Starting Good to Great by Jim Collins next.
What’s the best thing a customer has ever said to you?
“You care about us.”
“We want people to love what they use/do each day”
Where do you get your support leadership news?
My mentor and leadership group, Thrive.
What words make your skin crawl?
“Moist.”
How would you explain your job to an alien?
I make online experiences and digital products better for people.
What’s your most-used emoji in customer chats?
🎉
Do you identify more with the title “customer support,” customer service,” “customer success,” or “customer experience,” and why?
“Customer experience” – we want people to love what they use/do each day.
What was your “15 minutes of fame” moment?
I had to blow fire, topless, at a nighttime safari in Singapore in front of hundreds of people. Almost went viral for nearly 15 minutes.
What do you wish people knew about working in customer service?
We care.
Conversation closed… for now 😏
If you’re interested in being featured in our Response Time series, you can share your insights on customer service – and which celebrity would be great at your job – with us here.
Your first 30-60-90 days using AI for customer service
If you’re still the person thinking, “I’ll just wait and see how all of this AI stuff plays out,” it’s time to reconsider. AI is not going away. It’s not a fad.
The entire customer service industry is talking about this. Your customers are expecting it: 87% of support teams have seen an increase in their customers’ expectations over the last year, and 68% believe that expectations have been directly influenced by AI.
My advice? Just get started. Here’s the how, where, and when of rolling out AI for customer support in the first 30, 60, and 90 days (along with lessons we’ve learned at Intercom 👀).
Days 1-30: Set up and test AI
Start with an AI chatbot (or AI Agent)
First, set up an AI chatbot (or AI Agent) as a gateway to AI. Most businesses are already using some sort of chat. It’s an effective and easy way to introduce AI into the customer experience.
For example, trialing Intercom’s AI chatbot Fin AI Agent is a great way to get started. Using the most sophisticated AI language models, Fin automatically solves customer issues with safe, accurate, conversational answers based solely on your support content.
Trial AI with a small, trusted segment of customers
Pick a small, low-risk segment of customers to test your AI chatbot with. Most companies, especially in the B2B space, have a customer cohort of people who will be more amenable – maybe even excited – to try new things.
“We first tested AI with our early stage, startup customers”
At Intercom, we first tested AI with our early stage, startup customers. We knew that these were tech savvy customers who wouldn’t be scared to see AI popping up and asking them questions. That gave us some breathing room and goodwill as we tested answer qualities and found out what knowledge gaps we needed to fill.
Feed AI with the knowledge it needs
As our VP of Customer Support, Declan Ivory, says, “You must feed the AI beast with the knowledge it needs.” Start small and feed it with the help content you already have. If you’re using a complete AI-first customer service platform, like Intercom, Fin will be able to pull from multiple sources, including your:
- Public-facing help center content, PDFs, and URLs.
- Internal knowledge content, like Notion, Google Docs, or Confluence.
- A pool of your agent’s past conversations.
Even if you start with a low resolution rate at first, you’ll quickly be able to see where AI is answering customers’ questions versus where you have knowledge management gaps. Armed with these insights, you can make improvements in the next phase. 👇
Days 31-60: Beef up your knowledge base content
Fill in content gaps with your product team
By now, you’ll know where your chatbot is able to successfully resolve customer queries versus where it’s getting stuck. From there, you can create a plan to beef up your internal and external help content.
“We worked with teammates on the Product team to review relevant help articles and identify content gaps”
When we first rolled out our AI chatbot at Intercom, we had buy-in from the entire company. We worked with teammates on the Product team to review relevant help articles and identify content gaps. After the first 30 days, we were able to go back to those teams again and say, “Hey, we’re getting a lot of these types of questions. Fin is failing on these topics or on this part of the product area.” Then, they quickly jumped in and helped us add missing content.
Optimize your knowledge content for compounding returns
It’s worth going through your support content line by line. That may feel like a lot (we’re all strapped for time everywhere, especially in support). But the return on that investment is absolutely worth it because it’s going to give you compounding impact. The time you put in now will add up to all of these cases that your team don’t have to handle down the road, that you and your leadership team don’t have to manage. Big bonus: it will give your team breathing space to focus on solving more complex issues.
Days 61-90: Add AI value throughout the customer journey
Give your team their own personal assistant with an AI copilot
Once your AI chatbot is fine-tuned and performing well, look at the rest of the customer journey and identify where AI can add further efficiencies. For example, consider how AI can make your team more productive, make their lives easier, and help resolve customer queries faster.
A lot of companies have some version of an AI copilot in the inbox. When we rolled out Fin in the inbox (now rolled into Fin AI Copilot) in the first 90 days at Intercom, we thought, “How can we get our teammates using our AI inbox features more frequently?” Whether that’s summarizing a conversation or summing up a ticket when it’s being passed over, using AI in the backend can save teammates the hassle of scrolling all the way back up a conversation, rereading everything, and manually writing a message.
Since then, we’ve announced Fin AI Copilot – a personal AI assistant for every support agent. Now instead of wasting time searching for answers in the inbox or across numerous disconnected help docs, your support agents can ask Fin any question in the inbox and it will surface instant, accurate answers based on your support content.
Measure efficiency gains (you will see them)
An AI Copilot removes steps involved in troubleshooting issues and having to navigate a ton of different resources across multiple tools and docs. Early Fin AI Copilot beta customers are already seeing up to 31% efficiency gains across their team. Implementing AI chatbots in the right way will also lead to drastic resolution rate improvements, which enhances both the customer and teammate experience. Fin AI Agent resolves up to 50% of support queries instantly and accurately.
Bottom line: you’re going to see big efficiency gains. It’s important to set key benchmark metrics to get a regular pulse check on how AI is improving your support over time. You can use this data to get continuous buy-in from execs and your team on your AI-first customer service strategy. Once they see the positive impact AI is having for your customers, business, and team there’s no going back to the “old way.”
Don’t be afraid to pivot
Don’t be afraid to experiment and make changes as you learn new lessons. For example, at Intercom, we created a big triage flow where any questions Fin couldn’t answer, it would pass to a human. We thought this was going to be really valuable. In reality, it was a little cumbersome for customers. And it wasn’t adding much value to the support rep who was getting assigned that conversation. So we changed the flow to better serve customers and the team and it’s starting to work much better for everyone now.
The new age of customer service is AI-first
Despite all of the great progress we’ve made at Intercom, we still have plenty more to learn and explore to unlock AI’s full potential. We firmly believe the future of customer service is AI-first and we’re going all in on making that vision a reality. We’ll share our lessons as we build, test, learn, and iterate.
Embracing change, the customer service way
The impossible is now possible, or at least imaginable, with AI.
That’s what we regularly tell the leaders of the customer service teams using Intercom. For example, last year at Intercom we were aiming for an 8% resolution rate for our chatbots, and thought that it was a solid goal. Now our new target is 40% – a fivefold increase in a year!
Before, we would scarcely have believed that was achievable. But with advances in AI, everything has fundamentally changed. And so it’s important for us as support leaders to adopt a growth mindset. Here’s how we’re embracing change on the Intercom Customer Support team.
1. Stay open to, and seek out, possibilities
AI is impacting and accelerating everything, of course. But customer service is at the cutting edge of change – after all, OpenAI CEO Sam Altman has pointed to customer service as an area that is likely to see imminent disruption.
“To stay ahead, you have to be aware of how different things are and will continue to be”
The pace of development means that it’s hard to predict what things will look like by the end of the year, never mind two years from now.
For example, the idea that we could QA every conversation wasn’t possible before, but now we can do just that. To stay ahead, you have to be aware of how different things are and will continue to be. Stay open to and consistently seek out new possibilities.
2. Throw out your old assumptions and past learnings
One of our mantras over the past year has been to reconsider everything we thought we knew. We have to remind ourselves that, unless we’ve reviewed or discussed an area in the last 30-60 days, we need to go in with a blank slate.
“We’re in a different world now”
That’s how radically things have changed – throw away all of your past assumptions and past learnings, and start fresh.
Maybe we’ll still come to the same conclusions that we did a year ago, but we can’t rely on that institutional or historical knowledge because we’re in a different world now.
3. Help your team see the benefits of change
You’ll need your team on board too – it’s important to help them embrace change. Our advice? Talk directly to them about this big shift happening in support. Address any fears around AI and explain how these tools are not meant to replace them. Instead, help them see and experience the positives and possibilities of AI – how it helps them be more efficient, saves them time, and gives them more breathing room to take on more complex, meaningful work.
At Intercom, we firmly believe that the future of customer service is humans + AI working together to make customer service better. What’s possible for you today that wasn’t possible a year ago? The answer should give you a hint at the positive change to come.